Statistics in clinical and experimental medicine
Authors:
Jozef Rosina 1,2; Jiří Horák 3; Miluše Hendrichová 3; Karolína Krátká 3; Antonín Vrána 4; Jozef Živčák 5
Authors place of work:
Univerzita Karlova v Praze, 3. lékařská fakulta, Ústav lékařské biofyziky a lékařské informatiky, Česká republika
1; České vysoké učení technické v Praze, Fakulta biomedicínského inženýrství v Kladně, Česká republika
2; Univerzita Karlova v Praze, 3. lékařská fakulta, I. interní klinika FNKV, Česká republika
3; Univerzita Karlova v Praze, 2. lékařská fakulta, Česká republika
4; Technická univerzita v Košiciach, Strojnícka fakulta, Katedra biomedicínského inžinierstva a merania, Slovenská republika
5
Published in the journal:
Čas. Lék. čes. 2012; 151: 383-388
Category:
Přehledové články
Summary
The paper presents a brief overview of statistical methods used in clinical and experimental medicine, ranging from basic indicators and parameters of descriptive statistics and hypotheses testing (parametric as well as non-parametric methods) to a description of the most frequently multivariate methods used in medical scientific publications, to logistic regression. The paper also describes Principle Component Analysis (PCA), which is one of the methods used to decrease a data dimensionality. The proper use of statistical methods is demonstrated on specific clinical cases.
KEY WORDS:
Mean, median, t-test, Mann-Whitney U-test, ANOVA, Kruskal-Wallis ANOVA, Pearson’s chi-quadrat test, logistic regression, Principle Component Analysis
INTRODUCTION
Currently statistical analysis of medical data is above all a modeling-based science, a science searching for causes and relationships, a science evaluating risk factors for onset and development of diseases, etc. Since the first use of statistics medical research, or its first benefit to clinical medicine, it has become a tool without which modern clinical scientists often cannot present their results and conclusions or correctly interpret their claims.
When preparing a statistical evaluation, we acquire data by measuring or observing. Sometime the data is unclear or comes in large-volume at other times the data is scanty or incomplete. Only statistical analysis is able to transform these confusing collections of numbers which often, at first glance, overtly display no relationship or regularities into a set of tables, graphs and figures that can be discussed with the objective of formulating hypotheses and which we can share with colleagues as well as patients.
The clinician-scientist’s experimental activities as well as work are very demanding from a statistical point of view. Before clinicians (as well as experimental scientists) can see the results of their work, they sometimes have to wait several years as experiments are planned, research completed and data analyzed. Doctors must examine many patients as well as healthy individuals in order to acquire the data necessary to compare both groups, for confirming their expectations, etc. Awareness of this effort and labor teaches data analysts-statisticians to respect each number that a doctor presents to them.
Today modern medical information systems contain huge databases that are very complete, however, we still come across places where the data is lacking or incomplete, not only during retrospective studies but also in carefully planned prospective studies when only the statistical processing of data reveals new possibilities, inspires new hypotheses for doctors that will have to be tested and poses a number of new problems. Good statisticians should be able to select statistical methods that can extract relevant information even from incomplete data; moreover, they should be able to define additional requirements that enable researchers to find answers to new questions.
All of these aspects point to the need for close cooperation between clinicians and statisticians, not only during data processing itself, but from the very start in the study planning phase. It is also necessary for statisticians to understand the essence of the problem from a medical point of view, so that they can then better aid doctors in preparation of data collection requirements during creation of new studies. This understanding also make easier for statisticians to properly interpret correct results during data processing after the study is completed.
The existence of many high-quality professional statistics programs makes it possible for anyone to process data basically without any knowledge of statistical methods. This consequently leads to the danger of selecting and using incorrect methods for data processing, which could result in incorrect conclusions. That is why in this paper we will demonstrate, step-by-step and with very specific cases, several statistical methods – ranging from basic and simpler methods to more complicated methods. We will focus especially on the selection of the correct statistical method for a given medical issue and on the interpretation of results.
DATA ANALYSIS
The statistics is divided into two parts, i.e. descriptive statistics and inferential (or inductive) statistics with intention to use the findings gained from the sample of individuals to make inference about the relevant population. Inductive statistic, based on the probability theory, is objective and has the magic power of mathematical precisions (1).
The simplest outputs of statistical analysis are descriptive statistics (arithmetic means, standard deviations, medians, counts and percentages). More complicated methods include those that already belong to inductive statistics. There are hypotheses testing methods (t-tests, Mann-Whitney U-tests, parametric and non-parametric analysis of variance) and parameters which measure the strength of association of observed variables (Pearson and Spearman correlation coefficients). The most complicated analysis uses models of functional dependency, which are suitable e.g. for further predictions i.e. regression models, logistic regression, discriminant analysis.
Descriptive statistics
If we are describing what we observed or what we discovered in an observed set, without generalizing the results in any way, we can use the methods of descriptive statistics. We almost always use these methods before beginning any extensive statistical analysis in order to get information about the structure of the input data and to determine whether the input data meets the conditions of normal distribution (i.e. can we accept, based on the measured or observed values, the assumption of Gaussian distribution in relevant population). This finding then plays an important role in deciding whether a parametric (if the conditions for normality are met) or non-parametric (if the conditions for normality are not met) method will be needed for subsequent statistical analysis. The parametric methods can be used also in other probability distributions of known type, such as Poisson, binomial, multinomial, log-normal, exponential etc.
Descriptive statistical methods include frequency tables, absolute frequency, percentages, and indices, calculations of measures of central tendency (arithmetic mean, median, mode, etc.) and measures of spread (variance, standard deviation, range etc.), as well as graphs, which are used to present the results visually. These include, for example, histograms, scatterplots, range plots, means with error plots, box plots and many others.
We usually enter frequencies into frequency tables expressed in both absolute and percentage terms, which are among the simplest and most popular indicators in articles that are published in biomedical research (2 – 5). Frequency tables are commonly used as a basic method for evaluating results in the form of comparisons. We can compare our results to the results of other authors, to our own results obtained in previous studies under different conditions or to a previously someway established norm. In some cases, we can draw valid conclusions based on comparisons of absolute or percentage frequencies; however, if we are comparing results in percentages from sets of different sizes, we must always use one of hypotheses testing methods or risk introducing an error.
A good tool for comparing observation results for both absolute as well as percentage frequencies is a graphical depiction using a bar graph (6 – 9). This method for interpreting results is often much more clear than if we present the results merely as numerical values in tables.
The basic sample parameters of descriptive statistics include the arithmetic mean (average value) with standard deviation, or median, which are most frequently presented together with the range, i.e., a maximum and minimum value, or the 25% and 75% quartile. We commonly find examples of these basic characteristics in articles from biomedical research. We present studies (10 – 12) as examples. The statistician’s task is to decide which of the described parameters should be used and when the normality of the obtained data is a significant factor, since the arithmetic mean is best suited for homogenous sets. In the opposite case, when the sets are significantly non-homogenous (i.e., they contain extreme values, either very small or very large), the arithmetic mean provides a very distorted view about the observed variable, moreover it is also generally not true that half the set falls below the mean and half the set lies above the mean. A skewed distribution necessitates the use of additional statistical characteristics. The most suitable being the median, which really does divide the input data into to equal halves. It is insensitive to extreme values, which is why we also call it a robust estimate of the average value. The last significant parameter of descriptive statistics, especially for qualitative variables, is the mode, which is the value that appears most frequently in the sample. The mode is important, for example, in the evaluation of questionnaires and surveys, where we are interested in which response, to individual questions, appear most frequently (7, 13).
All of the mentioned above parameters belong to the point estimates, which are only the single numbers, calculated from available sample data. That is used to estimate the value of an unknown population parameter (but it says nothing about where this parameter really lies). More information brings the interval estimation which determines with given probability 1 – α the interval in which the real value of the unknown parameter will be found. The unknown parameter is estimated by two values LD and LH, which are the lower and upper limits of the confidence interval CI. The confidence interval will determine the position of the estimated unknown parameter of the distribution of the population with preselected, large enough probability P = 1 – α, which is called the coefficient of reliability or statistical certainty (commonly chosen 0.95 or 0.99). The parameter α is called the significance level (14).
Testing of hypotheses
At the start of a clinical study (clinical research) there is always a hypothesis, a statement related to a certain target group of patients. This statement is related to the observed variables in the target group – e.g. (i) values of biochemical tests, which we can compare with values from another group; this can involve, for example, a comparison of groups of patients with various diagnoses, or of patients and healthy control groups; (ii) measured values of diagnostics tests – a comparison of their results with the goal of determining whether the tests are equivalent (substitutable), or whether one of diagnostic methods is better; (iii) comparison of the frequency of responses in a questionnaire or survey from various groups of respondents, etc.
The hypothesis testing requires set up two competing statements – hypotheses. We recognize the null hypothesis (H0,), which we most often wish to reject or nullify with our study. This hypothesis is usually formulated as: “there is no difference in the observed variable between the groups of patients,” or “patients as well as the healthy control group belong to the same population from the perspective of the observed variable,” or “both diagnostics tests are equal,” etc. The second rival hypothesis is an alternative hypothesis (HA), which we would like to confirm with our results. It can be worded as follows: “there is a significant difference in the observed variable among the patient groups,” or “patients and healthy control groups belong to two different populations from the perspective of the observed variable,” or “the new diagnostics test is better than the current diagnostics method,” etc.
The research is thus supposed to either confirm or reject the given hypothesis. We can make two types of errors in this decision-making, specifically a type I error (α), where we reject the null hypothesis even though it is true, and a type II error (β), where we accept a null hypothesis that is in fact false. In order to make the correct decision about the null and alternative hypothesis and in order to avoid the two errors to the greatest degree possible (and the error that we want to avoid above all is the type I error), a number of statistical tests with their specific criteria are used. The most frequently used are the parametric tests and measures (characteristics): t-test, ANOVA (analysis of variance), Pearson’s correlation coefficient and non-parametric tests and measures: Mann-Whitney U-test, Kruskal-Wallis ANOVA and Spearman’s correlations. Non-parametric methods also include analysis of contingency tables, i.e. Pearson’s chi-squared tests or Fisher’s exact test. Then come methods that are much more complicated and in many cases multiple and multivariate, e.g. regression models, discriminant analysis, logistic regression, survival analysis and many methods that decrease the data dimensionality, i.e., reducing the number of variables, such as Principle Component Analysis (PCA), Factor Analysis, etc. In all of these methods hypotheses are constantly being tested.
It is necessary for the statistician to select the right statistical method, to decide correctly whether to use a parametric or non-parametric test, and conversely to also know when a parametric test can be used even if the condition of normality is not fulfilled, when to use a paired test and when an unpaired test, when a chi-squared test as well as the Mann-Whitney U-test can be used, to be able to convince a doctor that an ANOVA must be used instead of repeating t-tests several times, etc. It is thus apparent that a research team should include not only a doctor, but a statistician as well, especially when complicated statistical methods are expected to be used. His or her expertise and ability to decide on use of the optimal method is a guarantee that the obtained results will be evaluated as well as interpreted correctly.
Parametric vs. non-parametric tests
The basic condition for the use of parametric methods (t-test, ANOVA, Pearson’s correlations) versus non-parametric methods (Mann-Whitney U-test, Kruskal-Wallis ANOVA, Spearman’s correlations) is the normality of the distribution of the observed variables in all groups. Many methods are used to determine the normality of observed data – for example the Kolmogorov-Smirnov test, the Lilliefors adaptation of the Kolmogorov-Smirnov test, the Shapiro-Wilk test, etc. The results of these tests, however, are usually not presented in publications. Normality must be met especially for small samples, where N ≤ 15. In this case, it is always necessary to use non-parametric tests. Conversely, in cases where we have a large set of patients, i.e., N > 15, non-parametric tests are unsuitable since the tests do not work with real measured values, but only with ranks or with signs, etc., which results in considerable loss of information. Moreover, if a sample has a great size, it is possible to exclude the extreme data from the subsequent analysis.
The Mann-Whitney U-test must always be used if the data are discrete, or ordinal. In the last event it is necessary to assign numerical values – codes to the qualitative variables.
Mann-Whitney U-test vs. Pearson’s chi-squared test
The most frequently used method for analyzing observed qualitative variables is the analysis of contingency tables, where we assumed that each individual can be classified according two different factors (A and B, we observe the relative frequency of the incidence of a certain phenomenon – e.g., increased or normal value of systolic and diastolic pressure (A) in patients with differing diagnoses (B), in healthy and ill patients (B), in recovered patients or in those who died (B), in two groups of patients treated with two different methods (B), etc.), while each of the factors may have the different number of classes (or levels). After creating the contingency table (inclusion of all the individuals in the appropriate classes according to both factors) we may examine the relationship of both factors in (i) terms of stochastic independence (we examine whether the values of factor A do not affect the values of the factor B and vice versa) and (ii) testing the hypothesis of homogeneity (the relative frequency of individuals in each subpopulation (given by levels of factors A and B) are the same (or very similar) for all subpopulations (for all levels of factors A and B)) (15). For both cases the Pearson’s chi-squared or exact Fisher’s (in case of small sample size and only in 2x2 tables) tests are used.
We can see an example of the correct use of Pearson’s chi-squared test in study (16), where the goal was to compare the prevalence of the mutation of the HFE (human hemochromatosis protein) gene in porphyria patients versus a control group of patients. The results of Pearson’s chi-squared test showed a significantly higher incidence of the mutation of the HFE gene in porphyria patients. Another study (6) tracked the incidence of a curative effect as well as of adverse effects (loss of pigments, formation of a scar at application site) in the treatment of hemangioma using four different types of lasers. Based on a comparison it was possible to determine which of the lasers was the most suitable for treating a particular disease. The aim of the study (17) was to investigate whether single nucleotide polymorphisms (SNP) affect the clinical presentation and predispose to increased risk for postoperative adverse events in patients undergoing coronary artery bypass grafting surgery (CABG). In the next study (18), the mortality of women with a breast carcinoma was compared, specifically between women whose breast carcinoma was discovered during a screening (preventive) examination and women who presented with a perceptible breast carcinoma and the illness was only confirmed for them. In the study (19) the Pearson’s chi-squared test and Fisher’s exact test was used to determine erythrocyte phenotyping in blood donors and patients with sickle cell anemia (19) etc. It is clear from all of the clinical cases described above that an analysis using Pearson’s chi-squared test is suitable especially in cases where the observed variable is of a qualitative nature – presence of a mutation of gene (Yes/No), loss of pigment (Yes/No), patient dies (Yes/No), etc., i.e., where for the statistical analysis we only have counts of patients in individual categories of the observed variable.
To analyze qualitative data we can also use the Mann-Whitney U-test, specifically in cases where the qualitative data is the outcome of a scoring system, e.g., a comparison of histopathological grading for two different oncological diagnoses, the incidence and degree of genitourinary and gastrointestinal toxicity and its comparison among oncology patients treated with two different radiation techniques (20). Pearson’s chi-squared test (analysis using contingency tables) would have been the first choice method, but we can transform the scoring grading to numerical codes and use the Mann-Whitney U-test, which according to several studies (21) is far more sensitive, i.e., has a greater ability to differentiate among patient groups than Pearson’s chi-squared test.
We must be very careful in using the Mann-Whitney U-test in ordinal and qualitative data (after assigning numerical values – codes). We must pay attention that the differences between the stages (levels) of the scoring system (e.g. degree of differentiation, extent of damage, extent of disorders) were at least approximately the same.
ANOVA vs. t-test (resp. Kruskal-Wallis ANOVA vs. Mann-Whitney’s U-test)
Clinicians frequently make the mistake of comparing observed variables among three and more groups of patients, when they want to “completely logically” use a repeated t-test or non-parametric U-test successively between all pairs of groups. Here it is up to the statistician to explain to the doctor the growing type I error when a t-test is repeated on the same set of data (explanation of this growth is beyond the scope of this paper). We can see the correct use of the non-parametric Kruskal-Wallis analysis of variance and non-parametric correlation analysis (Spearman’s correlation coefficient) in study (22). This study examined gene expression of duodenal iron transport molecules and hepcidin in patients with hereditary hemochromatosis (HHC) (treated and untreated), involving various genotypes (genotypes which represent risk for HHC were examined), and in patients with iron deficiency anemia (IDA).
The correct procedure for analysis of variance is as follows: in the first step we perform an analysis of variance and if the test is statistically significant we perform multiple comparison tests, so called post-hoc tests: LSD (least significance difference), Scheffe’s test, Bonferroni’s test, etc. Currently, several authors (21, 23, 24) claim that it is not necessary to perform an analysis of variance and that it is possible to proceed with multiple comparison tests right away. This method should reduce the likelihood of making type II errors.
Parametric vs. non-parametric correlations
Many papers examine the relationship between two variables measured on objects (patients) entering the study. Here, once again, depending on whether the measured variables are quantitative and normal, or – to opposite – the values are discrete and ordinal (coded into numerical equivalents) and thus do not fulfill the conditions of normality, we either calculate a parametric correlation coefficient (Pearson’s), or a non-parametric coefficient (Spearman’s). Pearson’s correlation coefficient is used to determine the degree of the linear relationship between observed variables. Spearman’s correlation tells us that a pair of measured values lies on an ascending or descending function. Both correlation coefficients express only the functional dependence of observed variables; they say nothing about the causality. Found the relationship between variables may indicate that: (i) there is indeed causal relationship between variables; (ii) there exists the third variable that affects the both the dependent and influencing variable – apparent association and (iii) the observed relationship arises only due to coincidence – random variation. Examples of correlation analysis are presented in many studies (25 – 30).
Multivariate methods
The most complicated methods are multivariate statistical procedures, which try to describe the change of one or more variables as a consequence of the change of one or more other variables. The most common are the methods, where on the one hand we have one output, dependent variable and on the other hand many input, independent influencing variables, which can be of any type – continuous, discrete, qualitative, etc. These methods are called multivariate.
If the input variable is continuous and we are interested in how this variable changes due to the influence of the input variables, we can use general linear or non-linear models, general regression models, etc. For classification purposes, i.e., to find input variables that somehow influence the classification of patients or respondents into two or more groups, we use, in the event of continuous influencing factors that also meet the condition of normality, discriminant analysis. If the normality condition is not met, an alternative to this method – logistic regression can be used, which is also the most frequently used multivariate method in biomedical research.
Logistic regression
Logistic regression was proposed in the 1960’s as an alternative to the Discriminant analysis for cases where the variable being explained is binary, dichotomous. In clinical medicine, this output variable can assume, for example, the following values: patient lives or died, presence or absence of illness, remission or relapse of an illness, etc. Presently, with the existence of many professional statistical programs, the output variable can also assume multiple states. According to its character, we can, in addition to binary, also speak of ordinal or nominal logistic regression. Independent influencing variables can be of any type: continuous, discrete, or categorical. A logistic model reveals whether independent variables differentiate sufficiently well between individual classes (or states), which are defined by the output variable. In many cases it is used to predict a certain phenomenon (whether it occurred or not) and it is even able to say which of the given input variables had the most influence.
In order to assess whether a logistic model is statistically significant and also whether its classification and discriminatory ability is sufficient, there are a number of tests that must be calculated and correctly interpreted after the logistic model is created. The characteristics that evaluate and determine the statistical significance of a logistic model are: (i) deviance or (“negative two log likelihood”) test –2LL, which was used to judge the overall fit of a model. Using the deviance we may compare the models as well as with their difference, which represents the change of the goodness of fit from one model to another. The difference in deviance (so called likelihood ratio) is defined as follows: first we calculate the deviance for the model with just the intercept and then the deviance for model with selected groups of predictors. The difference between these deviances is asymptotically equal to χ2 distribution and tests the null hypothesis H0: “All regression coefficients are zero” against the alternative HA: “At least one regression coefficient is different than zero”– it tells or indicates whether the created logistic model is better than a model containing only the intercept (initial model); (ii) Wald’s criterion, which tests the statistical significance of each coefficient; (iii) Hosmer-Lemeshow test of goodness of fit, which tells us whether the model that we have created interpolates data well or not. For logistic regression it can happen that a model is statistically significant but it does not have to have any practical significance for classification purposes. The following tools are used to assess a model’s predictive and classification abilities: (i) classification table, which compares the model predicted and actually observed classification into classes of output variables; (ii) area under the ROC (Receiver Operating Characteristic) curve – we can find an exact interpretation of the size of this area in (31, 32).
It is important to verify all statistical analysis models that serve as the classification of objects, i.e., determine whether the created model actually categorizes the individual elements (patients) into the right classes. We can verify the model using the same data set, but much greater value is to verify it on completely different (new) data that was not used in the model’s creation. However, we almost never encounter this type of verification in medical publications.
We find logistic regression in many publications (33). In paper 33 we see not only the use of a logistic model in differentiating between benign and malignant tissues relative to colorectal carcinoma via the autofluorescence method (we define autofluorescence as the ability of various tissues to spontaneously emit light of various wavelengths – always in a certain spectrum), but also the confirmation of the discriminatory power of the created model on a set of histological samples (these samples were not used in the creation of the logistic model). Moreover, in this study we also see use of PCA for determining the wavelengths of radiation, which have a decisive significance for the diagnosis.
Principle Component Analysis (PCA)
The final method presented will introduce one of the oldest and most frequently used methods of multivariate analysis: Principle Component Analysis.
In Principle Component Analysis, attributes are not divided into dependent and independent variables as in regression. The method’s main principle consists of the linear transformation of the original attributes to new uncorrelated variables – principle components. The basic characteristic of each principle component is its degree of variability or variance. Because the principle components are ordered according to importance, i.e., in descending variance (from the largest to the smallest), the greatest amount of information about the variability of the original data is concentrated in the first principle component, and the least information is then concentrated in the last component. The standard use of PCA is to decrease the data dimensionality with a minimum loss of information.
The utilization of Principle Component Analysis is seen in the above mentioned study 33. From 200 various wavelengths of emitted autofluorescent spectrum radiation of benign and malignant tissue, the authors selected 30 and 40 respectively, as the most important wavelengths for differentiating between sick and healthy tissue. These wavelengths were subsequently used to create a logistic regression model.
CONCLUSION
Our paper has presented the reader, particularly clinicians, a view of some statistical methods used in biomedical research and in clinical studies, which are frequently encounter published papers, starting with some of the simplest ones and extending all the way to of the most complex, multivariate ones. Our goal was for the reader to become familiar with these statistical methods, so that they can correctly read and analyze results from medical publications. Additionally, we want the reader to be able to decide him or herself which of the possible statistical analysis methods they should use as they consider their own research. Our article showed the difference between parametric and non-parametric methods and also explained the necessity of using analysis of variance (ANOVA) in cases when comparing an observed variable among more than two groups of patients. Lastly we presented an overview of multivariate statistical methods, which are frequently used in medical publications, logistic regression.
Abbreviations
ANOVA | analysis of variance |
HFE | human hemochromatosis protein |
HCC | hereditary hemochormotosis |
IDA | iron deficiency anemia |
LSD | least significance difference |
ROC | receiver operating characteristic |
PCA | principle component analysis |
SNP | single nucleotide polymorphism |
CABG | coronary artery bypass graft |
Address of the corresponding author:
Doc. MUDr. Jozef Rosina, Ph.D.
Ústav lékařské biofyziky a lékařské informatiky
Univerzita Karlova v Praze
Ruská 87
100 00 Praha 10
e-mail: jozef.rosina@lf3.cuni.cz
tel.: 00420 267 102 305
Zdroje
1. Zvárová J. Biomedicínská statistika I. Praha: Karolinum 2011.
2. Majumdar A, Singh TA. Comparison of clinical features and health manifestations in lean vs. obese Indian women with polycystic ovarian syndrome. J Hum Reprod Sci 2009; 2(1): 12–17.
3. Vogl TJ, traub R, ichler K, oitaschek D, ack MG. Malignant liver tumors treated with MR imaging-guided aser-induced thermotherapy: experience with complications in 899 patients (2,520 lesions). Radiology 2002; 2): 367–377.
4. Remlová E, Vránová J, Rosina J, Navrátil L. nalysis of therapeutical effects of Er:YAG and CO aser post treatments of small hemangiomas. Laser Physics 2011; 21(9): 1665–1669.
5. Slavíčková R, Monhart V, Žabka J, Suchanová J, Ryba M, Peiskerová M, Trojánková M, Zahálková J, Sobotová D, Horáčková M, Ságová M, Jirovec M, Hajný J, Vránová J, Dusilová-Sulková S. Anemia and iron metabolism in predialysis CKD 2–5 chronic kidney disease patients (Anémie a metabolismus železa u nemocných dispenzarizovaných pro chronické onemocnění ledvin Stadia 2–5). Aktuality v Nefrologii 2009; 15(2): 53–62.
6. Remlova E, Dostalová T, Michalusová I, Vránová J, Navrátil L, Rosina J. Hemangioma curative effect of PDL, alexandrite, Er:YAG and CO2 asers. Photomedicine and Laser Surgery 2011; 29(12): 815–825.
7. Vranova J, renbergerova M, renberger P, tanek J, rana A, ivcak J, osina J. Incidence of cutaneous malignant melanoma in the Czech Republic: The risks of sun exposure for adolescents. Neoplasma 2012; 59: 316–325, Feb 2:1-2. doi: 10.4149/neo_2012_041 (Epub ahead of print).
8. Hill S, pink J, adilhac D, dwards A, aufman C, ogers S, yan R, onkin A. Absolute risk representation in cardiovascular disease prevention: comprehension and preferences of health care consumers and general practitioners involved in a focus group study. BMC Public Health 2010; 10: 108.
9. Elting LS, artin CG, antor SB, ubenstein EB. Influence of data display formats on physician investigators‘ decisions to stop clinical trials: prospective trial with repeated measures. BMJ 1999; 318(7197): 1527–1531.
10. Yeh EA, einstock-Guttman B, amanathan M, amasamy DP, illis L, ox JL, ivadinov, R. Magnetic resonance imaging characteristics of children and adults with paediatric-onset multiple sclerosis. Brain 2009; 32(Pt 12): 3392–3400.
11. McMahon LP, ent AB, err PG, ealy H, rish AB, ooper B, ark A, oger SD. Maintenance of elevated versus physiological iron indices in non-anaemic patients with chronic kidney disease: a randomized controlled trial. Nephrol Dial Transplant 2010; 5(3): 920–926.
12. Málek F, Havrda M, Fruhaufová Z, Vránová J. Short-term effect of evidence-based medicine heart failure therapy on glomerular filtration rate in elderly patients with chronic cardiorenal syndrome. Journal of the American Geriatrics Society 2009; 57(12): 2385–2386.
13. Finestone A, chlesinger T, mir H, ichter E, Milgrom C. Do physicians correctly estimate radiation risks from medical imaging? Arch Environ Health 2003; 58(1): 59–61.
14. Meloun M, Militký J. Statistická analýza experimentálních dat. Praha: Academia 2004.
15. Kubánková V, Hendl J. Statistika pro zdravotníky. Praha: Avicenum 1986.
16. Kratka K, Dostalikova-Cimburova M, Michalikova H, Stransky J, Vranova J, Horak J. High prevalence of HFE gene mutations in patients with porphyria cutanea tarda in the Czech Republic. British Journal of Dermatology 2008; 159(3): 585–590.
17. Emiroglu O, urdu S, gin Y, kar AR, lakoc YD, aim C, zyurda U, kar N. Thrombotic ene olymorphisms and postoperative outcome after coronary artery bypass graft surgery. J Cardiothorac Surg 011; 6: 120.
18. Bílková A, Zemanová M, Janík V, Vránová J. Comparison of women‘s mortality of breast cancer identified in the screening and diagnostic examinations (Porovnání úmrtnosti žen na karcinom prsu zjištěným při screeningovém a diagnostickém vyšetření). Ces Radiol 2011; 65(4): 272–278.
19. Pinto PC, raga JA, antos AM. Risk factors for alloimmunization in patients with sickle cell nemia. Rev Assoc Med Bras 011; 57(6): 668–673.
20. Vranova J, Vinakurau S, Richter J, Starec M, Fiserova A, Rosina J. The evolution of rectal and urinary toxicity and immune response in prostate cancer patients treated with two three-dimensional conformal radiotherapy techniques. Radiat. Oncol 2011 27; 6: 87.
21. Kobayashi K, Pillai KS, Sakuratami Y, Takemaru A, Kamata E, Hayashi M: Evaluation of statistical tools used in short-term repeated dose administration toxicity studies with rodents. J. Toxicol. Sci. 2008; 33(1): 97–104.
22. Dostalikova-Cimburova M, ratka K, alusikova K, hmelikova J, ejda V, nanicek J, eubauerova J, ranova J, ovar J, orak J. Duodenal expression of iron transport molecules in patients with hereditary hemochromatosis or iron deficiency. J Cell Mol Med 011. doi: 10.1111/j.1582‑4934. 2011.01458.x (Epub ahead of print).
23. Hamada C, Yoshino K, Matsumoto K, Nomura M, Yoshimura I. Three type algorithm for statistical analysis in chronic toxicity studies. J. Toxicol. Sci. 2008; 23: 173–181.
24. Kobayashi K, Kanamori M, Ohori K, Takeuchi H: A new decision tree method for statistical analysis of quantitative data obtained in toxicity studies on rodents. San Ei Shi 2000; 42: 125–129.
25. Long AC, ‘Neal HR Jr, eng S, ane KB, ight RW. Comparison of pleural fluid N-terminal pro-brain natriuretic peptide and brain natriuretic-32 peptide levels. Chest 2010; 137(6): 1369–1374 (Epub 2010 Feb 5).
26. Andrade H, orillas P, astillo J, oldán J, ateo I, gudo P, uiles J, ertomeu-Martínez V. [Diagnostic accuracy of T-proBNP ompared with electrocardiography in detecting left ventricular hypertrophy of hypertensive origin]. Rev Esp Cardiol 011; 64(10): 939–941 (Epub 2011 Jun 12).
27. Kim WS, ark SH. Correlation etween N-Terminal Pro-Brain Natriuretic Peptide and Doppler Echocardiographic Parameters of Left Ventricular Filling Pressure in Atrial Fibrillation. J Cardiovasc Ultrasound 011; 19(1): 26–31 (Epub 2011 Mar 31).
28. Vondráková D, Málek F, Ošťádal P, Vránová J, Miroslav P, Schejbalová M, Neužil P. Correlation of NT-proBNP, proANP and novel biomarkers: Copeptin and proadrenomedullin with LVEF and NYHA in patients with ischemic CHF, non-ischemic CHF and arterial hypertension. International Journal of Cardiology 2011; 150(4): 343–344.
29. Hendrichová M, Málek F, Kopřivová H, Vránová J, Ošťádal P, Krátká K, Sedláková M, Horák J. Correlation of NT-proBNP with metabolic liver function as assessed with 3C-methacetin breath test in patients with acute decompensated heart failure. International Journal of Cardiology 2010; 144(2): 321–322.
30. Ředinová-Vokrojová M, Šach J, Součkova I, Baráková D, Vránová J, Kuchynka P. The correlation between echographic and histopathological findings in uveal melanoma. Neuroendocrinology Letters 2008; 29(4): 536–546.
31. Vránová J, Horák J, Krátká K, Hendrichová M, Kovaříková K. Operating characteristic analysis and tne cost - benetit analysis in determination of the optimal cut-off point (ROC analýza a využití analýzy nákladů a přinosů k určení Optimálního dělícího bodu. Čas. Lék. čes. 2009; 48(9): 410–415.
32. The Magnificent ROC, http://www.anaesthetist.com/mnm/stats/ roc/Findex.htm
33. Ducháč V, avadil J, ránová J, irásek T, Štukavec J, orák L. Peroperative optical autofluorescence biopsy-verification of its diagnostic potential. Lasers in Medical Science 2011; 26(3): 325–333.
Štítky
Adiktologie Alergologie a imunologie Anesteziologie a resuscitace Angiologie Audiologie a foniatrie Biochemie Dermatologie Dětská dermatologie Dětská gastroenterologie Dětská gynekologie Dětská chirurgie Dětská kardiologie Dětská nefrologie Dětská neurologie Dětská onkologie Dětská otorinolaryngologie Dětská pneumologie Dětská psychiatrie Dětská radiologie Dětská revmatologie Dětská urologie Diabetologie Endokrinologie Farmacie Farmakologie Fyzioterapie Gastroenterologie a hepatologie Genetika Geriatrie a gerontologie Gynekologie a porodnictví Hematologie a transfuzní lékařství Hygiena a epidemiologie Hyperbarická medicína Chirurgie cévní Chirurgie hrudní Chirurgie plastická Chirurgie všeobecná Infekční lékařství Intenzivní medicína Kardiochirurgie Kardiologie Logopedie Mikrobiologie Nefrologie Neonatologie Neurochirurgie Neurologie Nukleární medicína Nutriční terapeut Obezitologie Oftalmologie Onkologie Ortodoncie Ortopedie Otorinolaryngologie Patologie Pediatrie Pneumologie a ftizeologie Popáleninová medicína Posudkové lékařství Praktické lékařství pro děti a dorost Protetika Psychologie Radiodiagnostika Radioterapie Rehabilitační a fyzikální medicína Reprodukční medicína Revmatologie Sestra Sexuologie Soudní lékařství Stomatologie Tělovýchovné lékařství Toxikologie Traumatologie Urgentní medicína Urologie Laboratoř Domácí péče Foniatrie Algeziologie Zdravotnictví Dentální hygienistka Student medicínyČlánek vyšel v časopise
Časopis lékařů českých
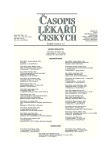
- Není statin jako statin aneb praktický přehled rozdílů jednotlivých molekul
- S profesorem Vladimírem Tesařem o léčbě CKD dle aktuálních doporučení: praktický lékař jako klíč k renoprotekci
- Porovnání empagliflozinu a dapagliflozinu v léčbě srdečního selhání
Nejčtenější v tomto čísle
- Hodnocení malnutrice hospitalizovaných dětí pomocí škály The Screening Tool for the Assessment of Malnutrition in Paediatrics
- Statistika v klinické a experimentální medicíně
- Transrektální sonografie prostaty u mužů s vrozeným hypogonadismem léčeným dlouhodobou substituční terapií testosteronem
- Marek Vácha, Radana Königová, Miloš Mauer: Základy moderní lékařské etiky