Genomics in Multiple Myeloma Research
Genomika ve výzkumu mnohočetného myelomu
Mnohočetný myelom je druhé nejčastější hematoonkologické onemocnění. Je to velmi heterogenní onemocnění charakterizované komplexitou genomu, opakujícími se amplifikacemi a/nebo delecemi, které vedou k rozdílnému klinickému projevu nemoci, ale i přežití u pacientů. Zejména nové metody genomiky hrají klíčovou roli v pochopení příčin patogeneze, progrese nemoci, ale i klasifikace MM.
Klíčová slova:
mnohočetný myelom – real-time PCR – SNP – GEP – patogeneze – prognóza
Tato práce byla podpořena granty MŠMT LC06027, MSM0021622434, granty IGA MZd NS10387, NS10406, NS10408 a GAČR GAP304/10/1395.
Autoři deklarují, že v souvislosti s předmětem studie nemají žádné komerční zájmy.
Redakční rada potvrzuje, že rukopis práce splnil ICMJE kritéria pro publikace zasílané do bi omedicínských časopisů.
Authors:
S. Ševčíková 1; P. Němec 1,2; L. Pour 3; R. Hájek 1,3,4
Authors place of work:
Babak Myeloma Group, Department of Pathological Physiology, Faculty of Medicine, Masaryk University, Brno, Czech Republic
1; Department of Experimental Biology, Faculty of Science, Masaryk University, Brno, Czech Republic
2; Department of Internal Medicine – Hematooncology, University Hospital Brno, Czech Republic
3; Laboratory of Experimental Hematology and Cell Immunotherapy, Department of Clinical Hematology, University Hospital Brno, Czech Republic
4
Published in the journal:
Klin Onkol 2011; 24(Supplementum 1): 34-38
Summary
Multiple myeloma (MM) is the second most common hematological cancer. It is a very heterogeneous disease characterized by large genomic complexity, recurrent amplifications and/or deletions in the genome leading to different clinical manifestations and survival of patients. Thus, genomics plays an important role in identifying agents responsible for pathogenesis, prognosis and disease stratification of MM.
Key words:
multiple myeloma – real-time PCR – SNP – GEP – pathogenesis – prognosis
This work was supported by projects of The Ministry of Education, Youth and Sports: LC06027, MSM0021622434; grants of IGA of The Ministry of Health: NS10387, NS10406, NS10408 and grant of Czech Science Foundation GAP304/10/1395.
The authors declare they have no potential conflicts of interest concerning drugs, products, or services used in the study.
The Editorial Board declares that the manuscript met the ICMJE “uniform requirements” for biomedical papers.
Introduction
New advances in genomics have made a great difference in understanding of various diseases, including multiple myeloma (MM). High-throughput genomic methods can help elucidate the entire transcription process – from aCGH analysis of the entire genome, to GEP array analysis of RNA transcripts, exon arrays of RNA splicing, miRNA arrays of miRNA, all the way to proteomics and post-translational modifications of proteins [1]. These methods are a valuable tool for prediction of outcome of MM patients.
In the field of MM, several studies have been published concentrating on changes at transcriptional level using gene expression profiling (GEP). One of the first ones was a study comparing MGUS and MM patients that suggested molecular basis for transformation from normal to malignant plasma cells [2]. Another study identified 4 subgroups of MM based on their similarity to MM cell lines [3]. Later, another study divided MM into 8 different subgroups and identified dysregulation of cyclin D expression as the main event of MM pathogenesis [4]. This classification was redefined in 2006 identifying 7 subclasses of MM [5]. Although this classification has not been widely accepted just yet, it is obvious that there are subgroups in MM that might perhaps lead to better characterization of the disease and different treatments for each group.
A major effort at the University of Arkansas has identified a signature of 70 genes capable of predicting high--risk MM. The team further showed that a simplified list of 17 genes is capable of providing the same prognostic discrimination [6]. This model discriminates with unprecedented ability ‘high-risk’ disease. This high-risk profile was indeed enriched for genes located in chromosome 1. The IFM also demonstrated in an independent series of 250 patients that a set of 15 genes was able to identify the patients with the poorest prognosis. It is possible that RT-PCR or immunohistochemistry-based strategies can be used to derive clinically applicable prognostic models for the disease. Other markers could include proliferation index by GEP, centrosome index by GEP and cancer testis antigens [4,7,8]. It is important to note that there is a minimal overlap between these different proposed signatures. The ability of each one of these signatures to be used in different context of treatment and stage of MM is still being validated. Furthermore, it is conceivable that novel GEP derived signatures could be developed in the future and will better predict patient outcome. This will also be important and relevant for new MM therapies.
In our laboratory, there are currently several genomic techniques performed. Real-time PCR and GEP (gene expression profiling) are discussed in the following section of this Supplementum. Array--CGH (whole-genome hybridisation) and SNP analysis will be discussed in separate chapters of this Supplementum.
Real-time PCR in Multiple Myeloma Research
PCR (polymerase chain reaction) is a method amplifying a fragment of DNA of known sequence by three basic steps: denaturation, annealing and elongation using a heat-stable DNA polymerase. This method allows for duplication of the target sequence in each step. There is another modification of this method that allows for RNA to be used as the primary material using reverse transcriptase to transcribe RNA into cDNA and then to follow the regular protocol [9].
Although basic principles of PCR were published in 1971 by Kleppe et al [10], it was not until 1985 when Saiki et al [11] published a report about amplification of beta-globulin in sickle cell anemia that this method gained more publicity. Kary B. Mullis, who was awarded the Nobel Prize for chemistry in 1993, took it further and used thermostable DNA polymerase thus making PCR a more accessible method [12]. Although end--point PCR was a great advancement at the time, currently it is thought to be a method with low dynamic range as well as low sensitivity and specificity.
Higuchi [13] first used amplification and detection of DNA sequences in real--time by adding ethidium-bromide (EtBr) to the reaction to visualize PCR product. As EtBr binds to DNA and fluoresces when UV light is used, positive amplification is marked by increased fluorescence. The following year, this group introduced the ‘real-time PCR’ method [14] where continuous measurements of increasing EtBr intensity were done. Real-time PCR is based on monitoring fluorescent signal from individual cycles all the way to the end of the reaction; the amount of product can be used to determine the amount of starting material. Unfortunately, EtBr binds non-specifically to DNA duplexes and other structures, so other probes had to be used [9].
At this time, there are basically three possibilities of running real-time PCR: fluorescent probes, molecular beacons and Syber Green method. For our purposes, fluorescent probes seem to be ideal.
Pitfalls of Multiple Myeloma Research
Multiple myeloma is characterized by presence of aberrant plasma cells in the bone marrow. These cells express CD138 marker on their surface; this marker is used for separation of these cells. Procedures for cell separation are described elsewhere in this Supplementum and will not be discussed here.
For molecular analyses, the purity of the sorted cell population is important and usually needs to be over 90% [6]. Our institute is located in close proximity to the hospital, so in our conditions, we receive the samples very soon after sorting of MM cells. Even so, questions remain if the separated population of cells is sufficiently close to the original conditions in vivo as we hope them to be [15]. Recently, several reports and presentations at conferences dealt with this issue suggesting that manipulation of these cells changed gene expression of various genes [16; our own unpublished data].
We have tried several methods of isolating RNA from MM CD138+ cells. As they are metabolically not very active, getting mRNA of sufficient yield and quality is problematic. In our hands, we have decided to start isolating RNA from fresh, unfrozen cells directly after they are separated. We have optimized the isolation protocol and tested different isolation kits, but in the end we decided to use RNEasy mini kit (Qiagen, USA). At this point, we get about 3.5 μg of RNA per 600,000 cells. We measure absorbance at 260, 280 and 230 nm. The values of ratios 260/280 nm and 260/230 nm have to be over 1.5 for RNA to be used for further analyses.
For frozen samples, we had great difficulties obtaining RNA of sufficient purity; especially ratios 260/230 were below the requested range. Although we followed the protocol religiously, we were not able to obtain good results. Based on recommendations of Qiagen technical support, we altered our protocol – immediately after thawing the cell pellet, we add RLT buffer with β-mercaptoethanol at suggested concentration and leave it for 1 hr at room temperature with occasional vortexing. This has made a tremendous difference, the ratio has increased and we have not had any problems with RNA purity since.
As RNA is a very precious commodity for us, and we need to share it among research teams, we use only 100 ng for reverse transcription. At this point in time, we use High Capacity Reverse Transcription Kit for reverse transcription (Applied Biosystems). To ensure that there is no contamination by genomic DNA, we run ‘NRTC’ (no RT controls – same amount of RNA into reaction but no reverse transcriptase) as well as ‘NTC’ (no template controls – water is added instead of the samples).
We use real-time PCR to analyse 17 genes suggested for high-risk disease [6]. As we need to run these reactions and do not have enough RNA for all of them, we started using preamplification reaction to increase our starting material (TaqMan PreAmp, Applied Biosystems) based on manufacturer’s instructions. Although this kit is supposed to preamplify without bias, we performed the initial optimization (based on manufacturer’s instructions) of preamplified and non-preamplified gene expressions. We were able to prove that, at least in our hands, our panel of genes can be preamplified in the same reaction. Although it does not happen often, it is possible that genes are not preamplified in the reaction to the same extend, and thus results may be biased or completely distorted. We run 14 cycles of preamplification to obtain enough material to run at least two real-time PCR plates.
We run real-time PCR using gene expression assays from Applied Biosystems (TaqMan Gene Expression Assays). These assays are ready-to-use and validated either in silico or in vitro, have high specificity and sensitivity and in PCR reactions have very similar effectivity. Reporter dye for our chosen genes is FAM. All our reactions are run as duplexes with GAPDH (with VIC as reporter dye) as the endogenous control.
The new MIQE guidelines [17] recommend running experiments in biological multiplicates rather than commonly used technical triplicates. However, due to the fact that the amount of material was and still is very limited, the variability minimization was performed at both RT and PCR (technical) steps, assuming consistent variability in patients cohort compared to healthy controls.
As it may be quite difficult to get healthy bone marrow, we decided to use commercially available RNA isolated from healthy bone marrow (Clontech) to set baseline of gene expression in healthy tissue. This bone marrow was obtained from 3 healthy individuals and mixed. Purity and integrity of this material is sufficient for our purposes with both absorbance ratios A260/A280 and A260/230 ≥ 2.0 ± 0.1 and RIN > 7.
For analysis, we use relative quantification in comparison with the healthy bone marrow – ΔΔCt calculation. This analysis has been described in great detail elsewhere [18]. This approach expects equivalent efficacies of both reference and target genes (Eref = Etarget = 2). The efficacies for individual genes were assessed previously with E value approaching 2. Normalization to GAPDH was employed to minimize variability among experiments. GAPDH was selected on basis of previous experiments. Ratios of normalized relative expression were calculated as R = 2–ΔΔCt, where ΔCt means Cttarget-Ctref calculated for both control (calibrator) and analysed sample. For statistical evaluation, data were log transformed, means and SEM were calculated and back-transformed to visualization in plots. Statistical significance was calculated on log-transformed data using parametrical tests. We compare gene expression of our patients’ samples to healthy bone marrow as well as several MM cell lines.
Gene Expression Profiling (GEP)
Methodology
Gene expression microarrays have now been used for over a decade to measure the gene-wise amount of RNA transcribed in a cell [19]. The standard type of chip in such an analysis is the so-called 3’ microarray that measures gene expression. This type of array was used in pilot studies in MM. In contrast, the GeneChip® Human Gene 1.0 ST Array is the latest product in the family of Affymetrix exon expression arrays offering whole-transcriptom coverage, providing a more complete and more accurate picture of gene expression than 3’ based expression array designs. The Human Gene 1.0 ST Array is part of a complete solution for gene expression analysis that includes Ambion/Affymetrix Whole Transcript (WT) Sense Target Labeling and Control Reagents, fluidics and scanning instrumentation and basic analysis software. Figure 1 shows the workflow for the new WT assay.
Our Experience with GeneChip® Human Gene 1.0 ST
Sample Preparation and Total RNA Processing
The advantage of WT assay in MM lies in the ability to use smaller amounts of total RNA as input material. Samples with 50 ng total RNA (3ul aqueous solution as maximum) can be used as starting material for processing with Ambion WT assay. In our lab, we start with 250 ng of total RNA whenever possible. Total RNA is isolated from CD138+ cells sorted by magnetic activated cell sorter (MACS) utilizing QIAGEN RNeasy Mini Kit according to manufacturer’s protocol with slight changes. We optimised QIAGEN protocol for isolation of total RNA from 0.65 × 106 CD138+ cells sorted by MACS. We usually obtain total RNA with sufficient quantity & quality when only 350 μl of appropriate buffer (RLT, RW1 and RPE) is used. Similarly, we only use 350 μl of 70% EtOH for RNA precipitation. The yield of total RNA is usually about 4 μg which is enough for further microarray and PCR processing. To avoid RNA degradation and changes of expression though the process, it is strongly recommended to isolate total RNA immediately after MACS sorting. Samples with CD138+ purity > 85% (measured by flow-cytometry) are suitable for expression analyses. RNA concentration is measured by NanoDrop Spectrophotometer, and RNA integrity number (RIN) is calculated using the Agilent 2100 Bioanalyzer (RNA 6000 Nano Kit). Total RNA with absorbance ratio A260/A280 higher than 1.7 and with RIN higher than 7.5 is acceptable for further processing by Ambion WT Expression Kit.
Ambion WT assay workflow encompasses the following: Total RNA acts as a template for synthesis of single-stranded cDNA. Single-stranded cDNA is then converted to double-stranded cDNA. Antisense cRNA is synthesized and amplified by in vitro transcription (IVT) of the second-strand cDNA. cRNA is purified using magnetic beads, so enzymes, salts, inorganic phosphates and unincorporated nucleotides are removed from cRNA solution. We made slight changes in the step of purification of cRNA. To achieve sufficient quantity of cRNA, it is necessary to use fresh isopropanol; it is also strongly recommended to use abundant volume of isopropanol for DNA precipitation. We recommend 100 ul instead of 60 ul. At these conditions cRNA yield usually reaches about 20 μg. To avoid low concentrations of cRNA in solution, we elute cRNA into 25 μl of elution buffer (instead of recommended 40ul). Total of 10 μg of cRNA (in total volume of 22 μl) is required for 2nd-cycle cDNA synthesis. Sense-strand cDNA is synthesized by reverse transcription of cRNA using random primers. The sense-strand cDNA contains dUTP at a fixed ratio relative to dTTP. RNA template is degraded using RNase H leaving intact single-stranded cDNA. Second-strand cDNA is purified to remove enzymes, salts and unincorporated dNTPs. Purifying 2nd-cycle cDNA seems to be the bottleneck of the Ambion protocol. Although we have changed the protocol by reducing volume of elution buffer from 30 μl to 22 μl, we still observe insufficient yields of cDNA in 1/3 of cases (this issue is now being discussed with Ambion support.).
Total of 5.5 μg of single stranded cDNA is fragmented and labeled using The Affymetrix GeneChip® WT Terminal Labeling Kit. We follow the manufacturer’s protocol for all further steps. The Human Gene 1.0 ST Array is then washed and stained by Affymetrix fluidics station and scanned by Affymetrix Scanner.
Differential Gene Expression Analysis
In our pilot study, we try to analyse differentially expressed genes among two different groups of MM patients. We used Agilent GeneSpring GX 11.5 software for expression analysis. Intensity values (CEL files) were summarised using gene level ExonRMA16 algorithm with quantile normalization and baseline transformation to median of all samples. For quality control of scanned arrays, we used Principal component analysis, array intensity comparisons as well as checking intensities/presence of array controls, hybridization control probes and Poly-A controls on arrays visualised by Genespring and/or Affymetrix Expression Console. Differences among groups were compared using T-test with Benjamini-Hochberg multiple testing correction. Fold change and p-value for all genes were calculated and visualised on Volcano plot. Selected genes were clustered using hierarchical clustering algorithm with Euclidean similarity measure.
Acknowledgement
The authors wish to thank all patients and doctors of the Czech Myeloma Group without whom this work would be impossible. We would like to thank Drahomira Kyjovska and her team for cell separation by MACS, Jana Stossova and Ivana Buresova for cell separation by FACS, and all members of the Babak Research Institute for helpful discussion and support.
Prof.
MUDr. Roman Hájek, CSc.
Babak
Myeloma Group
Department
of Pathological Physiology
Faculty
of Medicine
Masaryk
University
Kamenice
5
625
00 Brno
Czech
Republic
e-mail:
r.hajek@fnbrno.cz
Zdroje
1. Munshi NC, Avet-Loiseau H. Genomics in multiple myeloma. Clin Cancer Res 2011; 17(6): 1234–1242.
2. Davies FE, Dring AM, Li C et al. Insights into multistep transformation of MGUS to myeloma using microarray expression analysis. Blood 2003; 102(13): 4504–4511.
3. Zhan F, Hardin J, Kordsmeier B et al. Global gene expression profiling of multiple myeloma, monoclonal gammopathy of undetermined significance, and normal bone marrow plasma cells. Blood 2002; 99(5): 1745–1757.
4. Bergsagel PL, Kuehl WM, Zhan F et al. Cyclin D dysregulation: an early and unifying pathogenic event in multiple myeloma. Blood 2005; 106(1): 296–303.
5. Zhan F, Huang Y, Colla S et al. The molecular classification of multiple myeloma. Blood 2006; 108(6): 2020–2028.
6. Shaughnessy JD Jr, Zhan F, Burington BE et al. A validated gene expression model of high-risk multiple myeloma is defined by deregulated expression of genes mapping to chromosome 1. Blood 2007; 109(6): 2276–2284.
7. Chng WJ, Santana-Dávila R, Van Wier SA et al. Prognostic factors for hyperdiploid-myeloma: effects of chromosome 13 deletions and IgH translocations. Leukemia 2006; 20(5): 807–813.
8. Chng WJ, Ahmann GJ, Henderson K et al. Clinical implication of centrosome amplification in plasma cell neoplasm. Blood 2006; 107(9): 3669–3675.
9. Bustin SA. A–Z of quantitative PCR. 5th ed. California: IUL Biotechnology Series, La Jolla 2004–2006.
10. Kleppe K, Ohtsuka E, Kleppe R et al. Studies on polynucleotides XCVL. Repair replication of short sythetic DNA’s as catalysed by DNA polymerases. J Mol Biol 1971; 56(2): 341–361.
11. Saiki RK, Scharf S, Faloona F et al. Enzymatic amplification of beta-globin genomic sequences and restriction site analysis for diagnosis of sickle cell anemia. Science 1985; 230(4732): 1350–1354.
12. Mullis KB, Faloona FA. Specific synthesis of DNA in vitro via a polymerase catalyzed chain reaction. Methods Enzymol 1987; 155: 335–350.
13. Higuchi R, Dollinger G, Walsh PS et al. Simultaneous amplification and detection of specific DNA sequences. Biotechnology 1992; 10(4): 413–417.
14. Higuchi R, Fockler C, Dollinger G et al. Kinetic PCR analysis: real-time monitoring of DNA amplification reactions. Biotechnology 1993; 11(9): 1026–1030.
15. Ely S. Using aspirates for multiple myeloma research probably excludes important data. Br J Haematol 2006; 134(2): 238–246.
16. Sorrig R, Hermansen N, Hother C et al. CD138+ cell separation affects cancer gene expression in human myeloma cell lines. Haematologica 2010; 95 (Suppl 2): 137.
17. Bustin SA, Benes V, Garson JA et al. The MIQE guidelines: minimum information for publication of qunatitative real-time PCR experiments. Clin Chem 2009; 55(4): 611–622.
18. Pfaffl MW. A new mathematical model for relative quantification in real-time RT-PCR. Nucleic Acids Res 2001; 29(9): e45.
19. Ramsay G. DNA chips: state-of-the art. Nat Biotechnol 1998; 16(1): 40–44.
Štítky
Dětská onkologie Chirurgie všeobecná OnkologieČlánek vyšel v časopise
Klinická onkologie
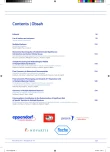
2011 Číslo Supplementum 1
- Metamizol jako analgetikum první volby: kdy, pro koho, jak a proč?
- Nejasný stín na plicích – kazuistika
- Neodolpasse je bezpečný přípravek v krátkodobé léčbě bolesti
- Příčiny a možnosti ovlivnění bolesti předního kolene po implantaci totální endoprotézy
- Léčba akutní pooperační bolesti z pohledu ortopeda
Nejčtenější v tomto čísle
- Multiple Myeloma
- Flow Cytometric Phenotyping and Analysis of T Regulatory Cells in Multiple Myeloma Patients
- Monoclonal Gammopathy of Undeterminated Significance: Introduction and Current Clinical Issues
- Flow Cytometry in Monoclonal Gammopathies