PREMATURE INFANT BLOOD VESSEL SEGMENTATION OF RETINAL IMAGES BASED ON HYBRID METHOD FOR THE DETERMINATION OF TORTUOSITY
Autoři:
Alice Krestanova 1; Jan Kubicek 1; Marek Penhaker 1; Juraj Timkovic 2
Působiště autorů:
VSB-Technical University of Ostrava, Ostrava, Czech Republic
1; Clinic Ophthalmology, University Hospital Ostrava, Ostrava, Czech Republic
2
Vyšlo v časopise:
Lékař a technika - Clinician and Technology No. 2, 2020, 50, 49-57
Kategorie:
Původní práce
doi:
https://doi.org/10.14311/CTJ.2020.2.02
Souhrn
The paper deals with the segmentation of the retinal vascular system using hybrid methods as morphological operations for the purpose of highlighting the extraction of blood vessels and tortuosity. Up to now tortuosity has been evaluated through a visual comparison of the retinal images. The output is an extracted retinal binary image with a blood vessel map. For this reason, a model was suggested that can automatically indicate the tortuosity of the retinal blood vessels by setting a threshold of the blood vessel curvature. This paper used a dataset of images (2800 images) from a RetCam3 device. Before applying the image processing, 30 images were selected with pre-plus diseases diagnosed, and this was divided into two groups with low contrast and higher contrast images. Part of the work is to determine the level of the tortuosity symptom by setting a threshold. Comparing the results with this processing method is not possible because the reference methods of image processing are based on fundus camera scanning, which has twice the resolution. This camera is not used for premature babies, but for children about one year of age and older or adults. Thus, retinal data for 14-day-old to 1-year-old children are not available for the fundus camera. This is a pilot study for the segmentation and mapping of blood vessels from retinal images taken by RetCam3.
Klíčová slova:
segmentation – retinal blood vessels – Curvature – tortuosity – RetCam3 – map vessels – hybrid methods
Zdroje
- Relan D, Ballerini L, Trucco E, MacGillivray T. Retinal Vessel Classification Based on Maximization of Squared-Loss Mutual Information. Advances in Intelligent Systems and Computing. 2016 Oct 2;390:77–84. DOI: 10.1007/978-81-322-2625-3_7
- Sodi A, Mucciolo DP, Murro V, Zoppetti C, Terzuoli B, Mecocci A, Virgili G, Rizzo S. Computer-assisted evaluation of retinal vessel diameter in retinitis pigmentosa. Ophthalmic Research. 2016;56(3):139–44. DOI: 10.1159/000446843
- Patwari MB, Manza RR, Rajput YM, Saswade M, Deshpande N. Automatic Detection of Retinal Venous Beading and Tortuosity by using Image Processing Techniques. IJCA Proceedings on National Conference on Recent Advances in Information Technology. 2014;2:203–7. DOI: 10.1109/ICICA.2014.51
- Pourezza HR, Pourezza M, Banaee T. Simple and efficient method to measure vessel tortuosity. ICCKE 2013. 2013;10: 219–22. DOI: 10.1109/ICCKE.2013.6682815
- Poletti E, Florin D, Grisan E, Ruggeri A. Automatic vessel segmentation in wide-field retina images of infants with Retinopathy of Prematurity. 2011 Annual International Conference of the IEEE Engineering in Medicine and Biology Society. 2011;8:3954–7, DOI: 10.1109/IEMBS.2011.6090982
- Onkaew D, Turior R, Kondo T, Uyyanonvara B. Automatic extraction of retinal vessels based on gradient orientation analysis. Computer Science and Software Engineering (JCSSE), 2011 Eighth International Joint Conference on: Piscataway: IEEE. 2011;6(8):102–7. DOI: 10.1109/JCSSE.2011.5930102
- Taševský P, Kolář R. Segmentace cévního řečiště ve foto-grafiích sítnice s využitím 2d diskrétní vlnkové transformace. Elektrorevue. 2009;48;4.
- Abbadi NK, Saadi EH. Automatic retinal vessel tortuosity measurement. Journal of Computer Science. 2013;9(11):1456–60. DOI: 10.3844/jcssp.2013.1456.1460
- Dougherty G, Johnson MJ, Wiers MD. Measurement of retinal vascular tortuosity and its application to retinal pathologies. Medical & Biological Engineering & Computing. 2010;48:87–95. DOI: 10.1007/s11517-009-0559-4
- Sil Kar S, Maity SP. Retinal blood vessel extraction using tunable bandpass filter and fuzzy conditional entropy. Computer Methods and Programs in Biomedicine. 2016;133:111–32. DOI: 10.1016/j.cmpb.2016.05.015
- Turior R, Chutinantvarodom P, Uyyanonvara B. Automatic tortuosity classification using machine learning approach. Applied Mechanics and Materials. 2012;241-244:3143–7. DOI: 10.4028/www.scientific.net/AMM.241-244.3143
- Sulik L, Krejcar O, Selamat A, Mashinchi R, Kuca K. Determining of blood artefacts in endoscopic images using a software analysis. Computational Collective Intelligence. 2015;9330:388–97. DOI: 10.1007/978-3-319-24306-1_38
- Cheng CS, Lee YF, Ong Ch, Yap C, Tsai ZL, Mohla A, Nongpiur ME, Aung T, Perera SA. Inter-eye comparison of retinal oximetry and vessel caliber between eyes with asymmet-rical glaucoma severity in different glaucoma subtypes. Clinical Ophthalmology. 2016;10:1315–21. DOI: 10.2147/OPTH.S96529
- Dziubek A, Guidobon G, Harris A, Hirani AN, Rusjan E, Thistleton W. Effect of ocular shape and vascular geometry on retinal hemodynamics: a computational model. Biomechanics and Modeling in Mechanobiology. 2016;15(4):893–907. DOI: 10.1007/s10237-015-0731-8
- Zhang S, She L, Ge X. Enhancement algorithms of retinal blood vessels based on curvelet conversion. Dongbei Daxue Xuebao/Journal of Northeastern University. 2016;37(7):922–6. DOI: 10.3969/j.issn.1005-3026.2016.07.003
- Blahuta J, Soukup T, Jelinkova M, Bartova P, Cermak P, Herzig R, Skoloudik D. A new program for highly reproducible automatic evaluation of the substantia nigra from transcranial sonographic images. Biomedical Papers. 2014;158:621–7. DOI: 10.5507/bp.2013.029
Štítky
BiomedicínaČlánek vyšel v časopise
Lékař a technika
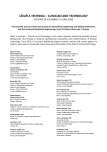
2020 Číslo 2
Nejčtenější v tomto čísle
- THE EFFECT OF FOOTWEAR TO THE POSTURE
- THE CHANGE OF CARDIOSTIMULATION DEVICE PROGRAMMING DUE TO DETECTION OF ELECTROMAGNETIC INTERFERENCE
- PREMATURE INFANT BLOOD VESSEL SEGMENTATION OF RETINAL IMAGES BASED ON HYBRID METHOD FOR THE DETERMINATION OF TORTUOSITY
- INAPPROPRIATE S-ICD PATIENT RECEIVES FALSE POSITIVE SHOCKS