Next generation sequencing: basic bioinformatic terms and analytic protocols for DNA analysis
Authors:
N. Tom 1,2; F. Pardy 3; J. Kotašková 1; K. Plevová 1,2; Š. Pospíšilová 1,2
Authors‘ workplace:
Centrum molekulární medicíny, CEITEC (Central European Institute of Technology), Masarykova univerzita, Brno
1; Centrum molekulární biologie a genové terapie, Interní hematologická a onkologická klinika, Fakultní nemocnice Brno a Lékařská fakulta Masarykovy univerzity, Brno
2; Centrální laboratoř Genomika, CEITEC (Central European Institute of Technology), Masarykova univerzita, Brno
3
Published in:
Transfuze Hematol. dnes,24, 2018, No. 3, p. 174-180.
Category:
Overview
Next generation sequencing (NGS) has become very popular both in research and clinical practice, in particular because it allows detailed and rapid insight into the patient's genome. Within the context of cancer research, NGS methods allow precise detection of germline and especially somatic mutations, which can help to diagnose a disease quickly and precisely and thus enable treatment administration based on individual patient needs. The development of novel computing methods and their application for accurate processing of NGS data is the objective of the scientific field of bioinformatics. Bioinformatic analysis is a complex process and its precise set-up is absolutely crucial for obtaining relevant results. Thus, it is necessary for bioinformaticians to understand the biological principles of the given analysis, such as the development of somatic mutations during disease course. From the perspective of a bio-analyst or physician, it is essential to understand the challenges and limits of NGS technology; basic knowledge of bioinformatics and its terminology allows for effective communication with bioinformaticians. In this review, the authors attempt to describe bioinformatic analysis with emphasis on explaining the basic concepts used in the NGS data analysis.
Key words:
next generation sequencing – bioinformatics – pipeline
Sources
1. Heather JM, Chain B. The sequence of sequencers: The history of sequencing DNA. Genomics 2016;107:1–8.
2. Tsiatis AC, Norris-Kirby A, Rich RG, et al. Comparison of Sanger sequencing, pyrosequencing, and melting curve analysis for the detection of KRAS mutations. J Mol Diagn 2010;12:425–432.
3. Malcikova J, Stano-Kozubik K, Tichy B, et al. Detailed analysis of therapy-driven clonal evolution of TP53 mutations in chronic lymphocytic leukemia. Leukemia 2015;29:877–885.
4. Xu C, Gu X, Padmanabhan R, et al. smCounter2: an accurate low-frequency variant caller for targeted sequencing data with unique molecular identifiers. bioRxiv 2018;281659; DOI: https://doi.org/10.1101/281659.
5. Newman AM, Lovejoy AF, Klass DM, et al. Integrated digital error suppression for improved detection of circulating tumor DNA. Nat Biotechnol 2016;34:547–555.
6. Gnirke A, Melnikov A, Maguire J, et al. Solution hybrid selection with ultra-long oligonucleotides for massively parallel targeted sequencing. Nat Biotechnol 2009;27:182–189.
7. Kivioja T, Vähärautio A, Karlsson K, et al. Counting absolute numbers of molecules using unique molecular identifiers. Nat Methods 2011;9:72–74.
8. Cock PJA, Fields CJ, Goto N, Heuer ML, Rice PM. The Sanger FASTQ file format for sequences with quality scores, and the Solexa/Illumina FASTQ variants. Nucleic Acids Res 2010;38:1767–1771.
9. Fabbro CD, Scalabrin S, Morgante M, Giorgi FM. An extensive evaluation of read trimming effects on Illumina NGS data analysis. PLoS One 2013; publ. elektronicky 23. 12. 2013. DOI:10.1371/journal.pone.0085024.
10. Fonseca NA, Rung J, Brazma A, Marioni JC. Tools for mapping high-throughput sequencing data. Bioinformatics 2012;28:3169–3177.
11. Sims D, Sudbery I, Ilott NE, Heger A, Ponting CP. Sequencing depth and coverage: key considerations in genomic analyses. Nat Rev Genet 2014;15:121–132.
12. Ebbert MTW, Wadsworth ME, Staley LA, et al. Evaluating the necessity of PCR duplicate removal from next-generation sequencing data and a comparison of approaches. BMC Bioinformatics 2016; publ. el. 25. 6. 2016. DOI:10.1186/s12859-016-1097-3.
13. Kou R, Lam H, Duan H, et al. Benefits and challenges with applying unique molecular identifiers in next generation sequencing to detect low frequency mutations. PLoS One 2016; publ. el. 11. 1. 2016. DOI:10.1371/journal.pone.0146638.
14. Xu C. A review of somatic single nucleotide variant calling algorithms for next-generation sequencing data. Comp Struct Biotechnol J 2018;16:15–24.
15. Zhao M, Wang Q, Wang Q, Jia P, Zhao Z. Computational tools for copy number variation (CNV) detection using next-generation sequencing data: features and perspectives. BMC Bioinformatics 2013;14:S1. publ. el. 13. 9. 2013. DOI:10.1186/1471-2105-14-S11-S1.
16. Smigielski EM, Sirotkin K, Ward M, Sherry ST. dbSNP: a database of single nucleotide polymorphisms. Nucleic Acids Res 2000;28:352–355.
17. International HapMap Consortium. The International HapMap Project. Nature 2003;426:789–796.
18. Forbes SA, Bhamra G, Bamford S, et al. The catalogue of somatic mutations in cancer (COSMIC). Curr Protoc Hum Genet 2008; publ. el. 6. 7. 2008. DOI: 10.1002/0471142905.hg1011s57.
19. Stenson PD, Mort M, Ball EV, Shaw K, Phillips AD, Cooper DN. The Human Gene Mutation Database: building a comprehensive mutation repository for clinical and molecular genetics, diagnostic testing and personalized genomic medicine. Hum Genet 2014;133:1–9.
20. Landrum MJ, Lee JM, Riley GR, et al. ClinVar: public archive of relationships among sequence variation and human phenotype. Nucleic Acids Res 2014; publ. el. 14. 11. 2013. DOI: 10.1093/nar/gkt1113.
21. Adzhubei I, Jordan DM, Sunyaev SR. Predicting functional effect of human missense mutations using PolyPhen-2. Curr Protoc Hum Genet 2013; publikováno el. 25. června 2015. DOI: 10.1002/0471142905.hg0720s76.
22. Robinson JT, Thorvaldsdóttir H, Winckler W, et al. Integrative genomics viewer. Nat Biotechnol 2011;29:24–26.
23. Babraham Bioinformatics – FastQC A quality control tool for high throughput sequence data. Dostupné na www: https://www.bioinformatics.babraham.ac.uk/projects/fastqc/. Accessed 15 Feb 2018.
24. Chen S, Huang T, Zhou Y, Han Y, Xu M, Gu J. AfterQC: automatic filtering, trimming, error removing and quality control for fastq data. BMC Bioinformatics 2017;18:80.
25. Schubert M, Lindgreen S, Orlando L. AdapterRemoval v2: rapid adapter trimming, identification, and read merging. BMC Research Notes 2016;9:88.
26. Martin M. Cutadapt removes adapter sequences from high-throughput sequencing reads. EMBnet.journal 2011;17:10–12.
27. Bolger AM, Lohse M, Usadel B. Trimmomatic: a flexible trimmer for Illumina sequence data. Bioinformatics 2014;30:2114–2120.
28. Li H, Durbin R. Fast and accurate short read alignment with Burrows–Wheeler transform. Bioinformatics 2009;25:1754–1760.
29. Langmead B, Salzberg SL. Fast gapped-read alignment with Bowtie 2. Nat Methods 2012;9:357–359.
30. Quinlan AR, Hall IM. BEDTools: a flexible suite of utilities for comparing genomic features. Bioinformatics 2010;26:841–2.
31. Broad Institute. picard: A set of command line tools (in Java) for manipulating high-throughput sequencing (HTS) data and formats such as SAM/BAM/CRAM and VCF. Dostupné na www: http://broadinstitute.github.io/picard. Accessed 22 Dec 2017.
32. Liao Y, Smyth GK, Shi W. featureCounts: an efficient general purpose program for assigning sequence reads to genomic features. Bioinformatics 2014;30:923–930.
33. Fulcrum Genomics. fgbio: Tools for working with genomic and high throughput sequencing data. Dostupné na www: https://github.com/fulcrumgenomics/fgbio. Accessed 15 May 2018.
34. Garrison E, Marth G. Haplotype-based variant detection from short-read sequencing. arXiv:12073907 [q-bio] 2012. Dostupné na www: http://arxiv.org/abs/1207.3907. Accessed 15 May 2018.
35. Van der Auwera GA, Carneiro MO, Hartl C, et al. From FastQ data to high confidence variant calls: the genome analysis toolkit best practices pipeline. Curr Protoc Bioinformatics 2013;11:11.10.1–11.10.33.
36. Lai Z, Markovets A, Ahdesmaki M, et al. VarDict: a novel and versatile variant caller for next-generation sequencing in cancer research. Nucleic Acids Res 2016; publ. el. 7. 4. 2016. DOI: 10.1093/nar/gkw227.
37. Koboldt DC, Zhang Q, Larson DE, et al. VarScan 2: somatic mutation and copy number alteration discovery in cancer by exome sequencing. Genome Res 2012;22:568–576.
38. Andrews TD, Jeelall Y, Talaulikar D, Goodnow CC, Field MA. DeepSNVMiner: a sequence analysis tool to detect emergent, rare mutations in subsets of cell populations. PeerJ 2016; publ. el. 24. 5. 2016. DOI: 10.7717/peerj.2074.
39. Abyzov A, Urban AE, Snyder M, Gerstein M. CNVnator: an approach to discover, genotype, and characterize typical and atypical CNVs from family and population genome sequencing. Genome Res 2011;21:974–984.
40. Medvedev P, Fiume M, Dzamba M, Smith T, Brudno M. Detecting copy number variation with mated short reads. Genome Res 2010;20:1613–1622.
41. Chen K, Wallis JW, McLellan MD, et al. BreakDancer: An algorithm for high resolution mapping of genomic structural variation. Nat Methods 2009;6:677–681.
42. Rausch T, Zichner T, Schlattl A, Stütz AM, Benes V, Korbel JO. DELLY: structural variant discovery by integrated paired-end and split-read analysis. Bioinformatics 2012;28:i333–i339.
43. Wang K, Li M, Hakonarson H. ANNOVAR: functional annotation of genetic variants from high-throughput sequencing data. Nucleic Acids Res 2010; publ. el. 3. 6. 2010. DOI: 10.1093/nar/gkq603.
44. Cingolani P, Platts A, Wang LL, et al. A program for annotating and predicting the effects of single nucleotide polymorphisms, SnpEff: SNPs in the genome of Drosophila melanogaster strain w1118; iso-2; iso-3. Fly (Austin) 2012;6:80–92.
Labels
Haematology Internal medicine Clinical oncologyArticle was published in
Transfusion and Haematology Today
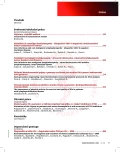
2018 Issue 3
Most read in this issue
- Non-infectious non-malignant lymphadenopathy – sinus histiocytosis with massive lymphadenopathy, Rosai-Dorfman disease
- Next generation sequencing: basic bioinformatic terms and analytic protocols for DNA analysis
- Recommendations for the diagnosis and treatment of chronic lymphocytic leukaemia (CLL) – 2018
- Non-infectious and non-malignant lymphadenopathy – idiopathic (HHV-8 negative) Castleman disease