Reconstruction and analysis of circRNA-miRNA-mRNA network in the pathology of lung cancer
Authors:
N. Hashemi 1; A. Jamshidian 2,3; S. Babaei 2,4; A. Khazraei-Monfared 2,4; A. Sayadi 2,5
Authors‘ workplace:
Department of Biology, Faculty of Biological Sciences, East Tehran Branch (Ghiamdasht), Islamic Azad University, Tehran, Iran
1; Biology Department, Kowsar poly-clinic, Tehran, Iran
2; Tehran Medical Genetics Laboratory, Tehran, Iran
3; Department of Cellular and Molecular Biology, Faculty of Biological Sciences, Islamic Azad University-Tehran North Branch, Tehran, Iran
4; Department of Cellular and Molecular Biology, Faculty of Biological Sciences, Islamic Azad University-Central Tehran Branch, Tehran, Iran
5
Published in:
Klin Onkol 2022; 35(6): 461-472
Category:
Original Articles
doi:
https://doi.org/10.48095/ccko2022461
Overview
Background: The presented study aimed to gain insights into the pathogenesis of lung cancer (LC) and provide novel biomarkers for LC by building a regulatory circular (circ) RNA‑micro (mi) RNA‑mRNA network. Materials and methods: High-throughput sequencing data of circRNAs, miRNAs and mRNAs related to LC originated from GEO (Gene Expression Omnibus) database, and the differential expressions of circRNAs, miRNAs and mRNAs were screened with R language Limma. The circRNA-miRNA and miRNA-mRNA pairs were used to build the ceRNA network. The functions of differential expression circRNAs were elucidated by performing the functional enrichment analysis on GO and KEGG. Furthermore, the selected LC prognostic genes were verified by tissue chips and immunohistochemistry (IHC). Results: On the whole, 20 downregulated circRNAs, 55 upregulated miRNAs and 243 downregulated mRNAs were identified in LC. Lastly, a circRNA-miRNA-mRNA ceRNA network was built, which was composed of 2 circRNAs, 2 miRNAs, and 2 mRNAs. As indicated from the analysis based on public databases and IHC, the differential genes (i.e., FXYD1 and SEMA5A) in this network acted as LC prognostic factors. As confirmed by performing IHC and survival analyses, FXYD1 and SEMA5A expressions in LC were downregulated, and their expressions displayed a relationship to the overall survival (OS) of LC cases. Conclusions: This study presents novel insights into the role of circRNAs in the development of LC via the ceRNA mechanism. The identified FXYD1 and SEMA5A gene could act as novel and vital LC prognostic indicators.
Keywords:
lung cancer – Prognosis – circular RNA – ceRNA network
Introduction
Lung cancer (LC) refers to one of the most common malignancies worldwide, and its morbidity and fatality have surged over the past few decades [1]. As suggested from the latest cancer statistics, the incidence and mortality of LC are the highest in malignant tumors globally [2]. Overall, the histological classification of LC can fall to two categories – non-small-cell lung cancer (NSCLC) and small cell lung cancer (SCLC) [3]. NSCLC takes up 80–85% in all LC, which is primarily split into adenocarcinoma and squamous cell carcinoma [3]. The prognosis of carcinoma displayed a close relationship to the period of clinical diagnosis: the postoperative 5-year survival rate after surgery was over 90% for patients at stage 0, about 70% of those at stage I, and patients at stages II–IV decreased from (30–40%) to (5–1%) [2–6]. However, nearly 75% of the patients with NSCLC were already in advanced stages at the time of admission, with a very low 5-year survival rate [3]. Accordingly, to improve the early diagnosis rate of LC and the survival rate, the related factors and molecular mechanisms of the occurrence and development of LC should be investigated in depth.
Over the past few years, circular RNA (circRNA) has attracted many attentions in the field of oncology. circRNA refers to a novel class of covalent closed-loop coding or non-coding RNAs, which were previously considered to be synthesized by mistranscription during the splicing process [7,8]. The lack of a 5‘ cap structure and a 3‘ poly A tail results in a long half-life and resistance to RNA enzymes that are expressed in a tissue-specific and developmental stage-specific manner [9]. circRNA has been confirmed to be extensively involved in gene transcription, post-transcription and even translation regulation [10]. It has been increasingly evidenced that abnormally expressed circRNAs can regulate tumor occurrence and development via the ceRNA mechanism: circRNA, the „molecular sponges“ of microRNA (miRNA), indirectly regulate the expressions of miRNA target genes by competitively binding to miRNA, which results in the abnormal expression of gene products (e. g., abnormal activation of proto-oncogenes) [11–13].
As sequencing technologies are advancing, RNA sequencing based on high-throughput platform has been adopted a tool to effectively excavate and identify novel transcripts [14]. Existing LC research based on ceRNA network remains in the preliminary stage. To screen out differentially expressed circRNAs, miRNAs and mRNAs, the authors acquired circRNA (GSE101684), miRNA (GSE53882) and mRNA (GSE116959) chip data related to LC from GEO database. On this basis, circRNA-microRNA and miRNA-mRNA pairs were screened, and circRNA-miRNA-mRNA gene networks were successfully built. Through GO analysis and KEGG function enrichment, in this network, mRNA molecular function and the main signaling pathways in the LC were analyzed. Furthermore, in TCGA database, the mRNAs associated with LC survival and prognosis were screened, and the differentially expressed genes were further verified by using tissue chips and IHC.
Materials and methods
Data collection
The LC gene chips required for analysis originated from the GEO database, including the original gene chips of human LC tissues and circRNA, miRNA and mRNA paired with non-tumor tissues. The circRNA gene chip data covered four pairs of LC tissues and their paired non-tumor tissues (GSE101684), the miRNA one consisted of 397 cases of LC tissues, 151 cases of paracancerous tissues (GSE53882), while the mRNA one involved 57 cases of LC tissues and 11 cases of normal lung tissues (GSE116959). By complying with the platform file of the chip data, the original probe matrix file was converted into circRNA matrix, miRNA matrix and mRNA matrix file, and the circRNA matrix was converted into the standard circBase name.
Differential analysis of circRNA, miRNA and mRNA and construction of ceRNA network
Bioconductor Limma packet of R language was adopted to read the initial data, and the background was corrected and then normalized. The difference analysis was conducted on the chip data. The differential expression RNA was screened, and the filtration condition was set as the absolute value of LogFold Change (FC) > 2, with the corrected P-value < 0.05. On that basis, differential circRNAs, miRNAs and mRNAs were obtained. Moreover, R language was employed to draw the heat map and volcano map of differential expression RNA for cluster analysis. By using the CSCD database, the structures of circRNAs and the target miRNAs bound by circRNAs were predicted. Next, the different mRNA was intersected with the target miRNA bound by circRNA, and the target mRNAs of the intersection of miRNAs were predicted online with miRDB and miRTARbase databases. In this study, only mRNAs (differential expression genes) identified by two databases were employed to intersect with the differentially expressed mRNAs obtained previously. Furthermore, the circRNA-miRNA-mRNA network was built and then visualized with Cyposcape software.
Functional enrichment analysis and survival analysis
To assess the potential biological functions of mRNA in the ceRNA network and the pathways involved, the “cluster profiler“ module of R software was exploited to conduct GO functional enrichment analysis and KEGG signaling pathway enrichment analysis on mRNA. GO analysis included three parts, i.e., molecular function, biological process and cell composition, and key pathways in LC development were obtained with KEGG database. The mRNA expression profile and clinical sample data regarding LC originated from the TCGA database, and the survival time and status of the patients were extracted, in combination with the mRNA data in the ceRNA network. Moreover, with LOGpc and Kaplan-Meier Plotter public databases, the value of differential expression genes (mRNAs) in the prognosis assessment of LC was analyzed. P < 0.05 indicated the statistically significant difference.
IHC and prognosis statistical analysis
Given the bioinformatics analysis data, tissue chip and IHC experiments were further performed to verify the expressions of differential genes in LC and the correlation with patient prognosis at the protein level. Then, the reliability of bioinformatics analysis data could be verified in depth. This study employed high-throughput breast cancer tissue microarray (HLugS180Su02, each tissue microarray containing 90 points of lung adenocarcinoma tissue and 90 points of corresponding paracancerous tissue) to analyze protein expression. By complying with the instructions of EnVision DAB test kit, the paraffin embedded tissues/cells fixed with 4 μM formaldehyde were analyzed by performing immunohistochemistry. PBS was used as negative control instead of primary antibody, and the light microscope (Olympus) was used to observe and take images. To verify whether the cells in the sections showed color and chromogenic degree, the scores included 0, 1, 2, and 3 for non-immune color, light brown, medium brown and dark brown, respectively. According to the positive staining proportion of sections, the score reached: < 5% for 0, 5–25% for 1, 25–50% for 2, and > 50% for 3, and the final score of protein expression is the product of score and intensity: 0 for −, 1–2 for +, 3–5 for ++, and 6–9 for +++, where ±/+ indicates low expression, and ++/+++ expresses high expression [15]. Given the clinical data and follow-up information of patients in the tissue chips, the Kaplan--Meier survival curve was plotted with GraphPad Prism 5.0 software to analyze the correlation between differential protein expression and patient prognosis. A cut-off value of P < 0.05 indicated the statistically significant difference.
Results
Screening of differentially expressed circRNAs, miRNAs and mRNAs
A total of 7 320 circRNAs, 1 842 miRNAs and 32 075 mRNAs related to LC were obtained in total from the GEO database. After threshold screening was conducted, this study found 24 differential circRNAs (20 downregulated), 108 differential miRNAs (55 upregulated), and 329 differential mRNAs (243 downregulated). Fig. 1 illustrates the basic information of circRNA (GSE101684), miRNA (GSE53882) and mRNA (GSE116959) in LC tissues and their pairing non-tumor tissues.
Visualization results of the ceRNA network
The basic information of the 24 differential circRNAs screened in the CSCD data was searched, and 16 circRNAs were identified. According to the prediction of this study, there might be 791 target miRNAs among the mentioned 16 circRNAs, and 20 intersection miRNAs were obtained by intersecting with differential miRNAs. Construction of the circRNA--miRNA-mRNA ceRNA network in LC has been shown in Fig. 2. Overall, 499 potential target genes were predicted with miTarbase and miRDB, and 10 intersecting mRNAs were obtained by intersecting them with a range of mRNAs. Lastly, Cytoscape software was employed to map a visual network of circRNA-miRNA--mRNA that was composed of two downregulated circRNAs, two upregulated miRNAs, as well as two downregulated mRNAs. Fig. 3 illustrates the heatmaps and boxplots of such circRNAs miRNAs and mRNAs involved in the network.
Enrichment analysis of functional and signaling pathway
As revealed from the results of GO analysis, the identified mRNAs showed the main enrichments in cellular response to extracellular stimulus, cellular response to external stimulus, and sulfur compound metabolic process for BP item (top 3), and caveola, plasma membrane raft and membrane raft for CC item (top 3), and MAP kinase activity, RNA polymerase II CTD heptapeptide repeat kinase activity, as well as mitogen−activated protein kinase binding. According to KEGG pathway analysis, the target gene showed a significant enrichment effect with the cAMP signaling pathway, terpenoid backbone biosynthesis as well as aldosterone−regulated sodium reabsorption (top 3), the graphical presentation of which has been demonstrated in Fig. 4.
Survival analysis and IHC validation
On the whole, 1,145 LC mRNA microarray expressing data and clinical sample data originated from the TCGA database. Two mRNAs (FXYD1, SEMA5A) in the built circRNA-miRNA-mRNA network were analyzed in depth. As manifested by GEPIA database, the expression of FXYD1 in both LUAD and LUSC LC subtypes was lower than that in the control. SEMA5A exhibited the consistent expression state with that of FXYD1. Moreover, as indicated from the prognostic analysis based on public databases, LC patients achieving high FXYD1 expression had longer overall survival time, which was suggested as a protective factor of patients’ prognosis. However, SEMA5A was not prognosis-significant in LOGpc database, whereas it was reported as a prognosis factor based on the analysis of Kaplan-Meier Plotter. As suggested by the IHC data, the expressions of FXYD1 and SEMA5A proteins in LC were downregulated (Fig. 5), and LC patients achieving a high expression of FXYD1 or SEMA5A were more likely to benefit from prognosis and overall survival (Fig. 6).
Discussion
CeRNA refers to a general term for a class of RNA molecules (e. g., mRNA, pseudogenes, lncRNA and circRNA) [16]. ceRNA reduces its inhibitory effect on mRNA translation by competitively binding to common miRNAs [11–13,17]. As confirmed by existing studies, dysregulation of ceRNA shows a tight relationship to the occurrence of a wide range of malignant tumors [17,18]. Based on the chip data of GEO database, this study identified the differential expression circRNAs, miRNAs and mRNAs, which have provided the foundation for the successful construction of a gene network. The mRNA molecular function and the main signaling pathways in the LC were analyzed by conducting GO and KEGG function enrichment analyses in this network. Furthermore, the mRNA associated with LC survival and prognosis was screened in TCGA database, and the prognostic significance of differential expression genes were verified in depth.
Based on the GEO database, this study selected microarray data of circRNA, miRNA and mRNA of LC and screened out 24 differential circRNAs, 108 differential miRNAs and 329 differential mRNAs. To more specifically study which circRNAs and their target genes (mRNAs) regulated via the ceRNA network are related to the prognosis of LC, this study built the circRNA-miRNA-mRNA regulatory network, and screened out the ceRNA regulatory axis that may be mediated by has_circ_0001806-miR-3178-FXYD1 and has_circ_0031968-miR-4270-SEMA5A. As proved by GO enrichment analysis, this network gene was involved in numerous important biological functions and metabolic pathways of LC (e. g., MAP kinase activity, cAMP signaling pathway and VEGF signaling pathway), which were closely related to the progression of LC.
Based on the TCGA database, ten differential mRNAs that were intersected were analyzed, and by using R language, a total of two mRNAs (FXYD1, SEMA5A) in this network were found to be associated with the prognosis of LC patients. GEPIA showed that FXYD1 and SEMA5A were downregulated in LC tissues. In addition, the expressions of FXYD1 and SEMA5A was positively correlated with the survival time of patients. There are few studies on the correlation of FXYD1 and a tumor. Reports like FXYD1 may be the important factors involved in the occurrence and development of gastric cancer [19]. This study initially reported that FXYD1 can achieve a low expression in both lung adenocarcinoma and squamous cell carcinoma, and a high expression of FXYD1 can act as a protective factor for the prognosis of LC patients. The study of SEMA5A in LC has been reported in some recent studies [20–22]. As reported by Lu TP et al, the downregulation of SEMA5A in tumor tissue at the transcriptional and translational levels was associated with poor survival among nonsmoking women with NSCLC [21]. Likewise, as further confirmed by a newly published study, the upregulation of SEMA5A significantly inhibited the proliferation, colony formation and migratory ability in A549 and H1299 lung adenocarcinoma cells, and hypermethylation of SEMA5A and the cleavage of the extracellular domain of SEMA5A contributed to the downregulation of the SEMA5A levels in such cell lines [20]. The expression and prognostic significance of FXYD1 and SEMA5A in LC by tissue microarray combined with IHC was further verified by our group, and FXYD1 and SEMA5A were found to achieve low expressions in LC. The expressions of FXYD1 and SEMA5A were the protective factors to conduct the prognosis of LC patients. Furthermore, the validation results here can confirm the reliability of the predictive analysis.
In this study, the LC expression profile gene chip of GEO database was employed to screen the differentially expressed circRNAs, miRNAs and mRNAs, the circRNA-miRNA-mRNA network was built, and the enrichment analysis of the functions and signaling pathways was conducted. The identified FXYD1 and SEMA5A genes can act as vital molecular markers for the prognosis of LC, the result of which has been demonstrated in Fig. 7. This study was also subject to many limitations (e. g., insufficient sample data and limited chip data combined analysis). Thus, the conclusion here should be verified by conducting considerable studies of higher quality.
Acknowledgements
This study was conducted at the Kowsar poly-clinic, Tehran, Iran, thus appreciating the spiritual support of this center is admitted.
Author contributions
Atefeh Sayadi and Dr. Shadi Babaei, the corresponding authors of this article, justify that all the mentioned individuals in this article are members of this research team and had had substantial contributions to conception and design, acquisition of data, analysis and interpretation of data, drafting the article, revising it, and final approval of the version to be published.
Consent for publication
All the authors confirm that the manuscript represents their honest work.
Availability of data and materials
The data used in this study are available from the corresponding author on request.
Arefeh Khazraei Monfared
Department of Cellular
and Molecular Biology
Faculty of Biological Sciences
Islamic Azad University – Tehran
North Branch
P.O. Box: 19585/466
Tehran, Iran
e-mail: dafili_p@yahoo.com
Mrs. Atefeh Sayadi
Biology Department
Kowsar poly-clinic
P.O. box: 1658143443,
Tehran, Iran
e-mail: movi_85@yahoo.com
Submitted/Obdrženo: 9. 1. 2022
Accepted/Přijato: 3. 5. 2022
Sources
1. Barta JA, Powell CA, Wisnivesky JP. Global epidemiology of lung cancer. Ann Glob Health 2019; 85 (1): 8. doi: 10.5334/aogh.2419.
2. Torre LA, Siegel RL, Jemal A. Lung cancer statistics. Adv Exp Med Biol 2016; 893: 1–19. doi: 10.1007/978-3-319-24223-1_1.
3. Blandin Knight S, Crosbie PA, Balata H et al. Progress and prospects of early detection in lung cancer. Open Biol 2017; 7 (9): 170070. doi: 10.1098/rsob.170070.
4. Li J, He J, Zhang Y et al. Survival in lung cancer among female never-smokers in rural Xuanwei and Fuyuan counties in Eastern Yunnan province, China. Zhongguo Fei Ai Za Zhi 2019; 22 (8): 477–487. doi: 10.3779/j.issn.1009- -3419.2019.08.01.
5. Zhou X, Zhang Z, Liang X. Regulatory network analysis to reveal important miRNAs and genes in non-small cell lung cancer. Cell J 2020; 21 (4): 459–466. doi: 10.22074/cellj.2020.6281.
6. Su T, He C, Li X et al. Association between early informed diagnosis and survival time in patients with lung cancer. Psychooncology 2020; 29 (5): 878–885. doi: 10.1002/pon.5360.
7. Meng S, Zhou H, Feng Z et al. CircRNA: functions and properties of a novel potential biomarker for cancer. Mol Cancer 2017; 16 (1): 94. doi: 10.1186/s12943-017-0663-2.
8. Zhang HD, Jiang LH, Sun DW et al. CircRNA: a novel type of biomarker for cancer. Breast Cancer 2018; 25 (1): 1–7. doi: 10.1007/s12282-017-0793-9.
9. Suzuki H, Tsukahara T. A view of pre-mRNA splicing from RNase R resistant RNAs. Int J Mol Sci 2014; 15 (6): 9331–9342. doi: 10.3390/ijms15069331.
10. Chen LL, Yang L. Regulation of circRNA biogenesis. RNA Biol 2015; 12 (4): 381–388. doi: 10.1080/15476286.2015.1020271.
11. Rong D, Sun H, Li Z et al. An emerging function of circRNA-miRNAs-mRNA axis in human diseases. Oncotarget 2017; 8 (42): 73271–73281. doi: 10.18632/oncotarget.19154.
12. Liang ZZ, Guo C, Zou MM et al. CircRNA-miRNA-mRNA regulatory network in human lung cancer: an update. Cancer Cell Int 2020; 20: 173. doi: 10.1186/s12935-020-01245-4.
13. Tian Y, Xing Y, Zhang Z et al. Bioinformatics analysis of key genes and circRNA-miRNA-mRNA regulatory network in gastric cancer. Biomed Res Int 2020; 2020: 2862701. doi: 10.1155/2020/2862701.
14. Pareek CS, Smoczynski R, Tretyn A. Sequencing technologies and genome sequencing. J Appl Genet 2011; 52 (4): 413–435. doi: 10.1007/s13353-011-0057-x.
15. Cui Z, Li Y, Gao Y et al. Cancer-testis antigen lactate dehydrogenase C4 in hepatocellular carcinoma: a promising biomarker for early diagnosis, efficacy evaluation and prognosis prediction, Aging (Albany NY) 2020; 12 (19): 19455–19467. doi: 10.18632/aging.103879.
16. Qi X, Zhang DH, Wu N et al. CeRNA in cancer: possible functions and clinical implications. J Med Genet 2015; 52 (10): 710–718. doi: 10.1136/jmedgenet-2015-103334.
17. Ergun S, Oztuzcu S. Oncocers: ceRNA-mediated cross-talk by sponging miRNAs in oncogenic pathways. Tumour Biol 2015: 36 (5): 3129–3136. doi: 10.1007/s13277-015-3346-x.
18. Chan JJ, Tay Y. Noncoding RNA: RNA regulatory networks in cancer. Int J Mol Sci 2018; 19 (5): 1310. doi: 10.3390/ijms19051310.
19. Zhang J, Liu X, Yu G et al. UBE2C is a potential biomarker of intestinal-type gastric cancer with chromosomal instability. Front Pharmacol 2018; 9: 847. doi: 10.3389/fphar.2018.00847.
20. Ko PH, Lenka G, Chen YA et al. Semaphorin 5A suppresses the proliferation and migration of lung adenocarcinoma cells. Int J Oncol 2020; 56 (1): 165–177. doi: 10.3892/ijo.2019.4932.
21. Lu TP, Tsai MH, Lee JM et al. Identification of a novel biomarker, SEMA5A, for non-small cell lung carcinoma in nonsmoking women. Cancer Epidemiol Biomarkers Prev 2010; 19 (10): 2590–2597. doi: 10.1158/1055-9965.EPI-10--0332.
22. Chen WC, Wang CY, Hung YH et al. Systematic analysis of gene expression alterations and clinical outcomes for long-chain acyl-coenzyme A synthetase family in cancer. PLoS One 2016; 11 (5): e0155660. doi: 10.1371/journal.pone.0155660.
Labels
Paediatric clinical oncology Surgery Clinical oncologyArticle was published in
Clinical Oncology
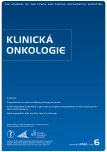
2022 Issue 6
Most read in this issue
- Prognostic and predictive factors of brain meningiomas
- Advantages and limitations of 3D organoids and ex vivo tumor tissue culture in personalized medicine for prostate cancer
- Fecal microbiota transplantation – new possibility to influence the results of therapy of cancer patients
- Fatal myocarditis after the first dose of nivolumab