Cooperation of Genomic, Transcriptomics and Proteomic Methods in the Detection of Mutated Proteins
Authors:
F. Zavadil Kokáš; J. Faktor; B. Vojtěšek
Authors‘ workplace:
Regionální centrum aplikované molekulární onkologie, Masarykův onkologický ústav, Brno
Published in:
Klin Onkol 2019; 32(Supplementum 3): 78-84
Category:
Review
doi:
https://doi.org/10.14735/amko20193S
Overview
Background: Current anti-tumour therapy is characterised by high non-specificity due to the diverse nature of tumours, which can significantly reduce its efficiency. The massive development of genomic, transcriptomic, and proteomic methods has enabled the detailed characterisation of individual tumours at the genome, transcriptome and proteome levels. Whole-genome sequencing, whole-transcriptome sequencing and exome sequencing can be listed as examples of genomics and transcriptomics methods. Those methods are suitable for detecting single-nucleotide polymorphisms. In the case of proteomic methods, where a peptide library is available, it is possible to detect mutated proteins in a biological sample. Also important is software that interprets and visualises the results or facilitates conversion between data formats that are specific to the method. The combination of methods can in principle increase the likelihood of detecting new neoantigens and design-specific anti-tumour therapy.
Aim: The article primarily describes the bioinformatics analysis of samples using the methods of genomics, transcriptomics and proteomics, and the possible problems which must be considered during the analysis. The article includes a description of TransPEM software designed to convert the results from the analysis of single nucleotide polymorphisms into a peptide library of sequences useful for the detection of neopeptides using proteomic methods. The publication is accompanied by a brief description of the proteomics methods using this peptide library and the summary of its limitations.
Keywords:
Genomics – proteomics – transcriptomics – bioinformatics – software development
Sources
1. Vucic EA, Thu KL, Robinson K et al. Translating cancer “omics” to improved outcomes. Genome Res 2012; 22(2): 188–195. doi: 10.1101/ gr.124354.111.
2. Conesa A, Madrigal P, Tarazona S et al. A survey of best practices for RNA-seq data analysis. Genome Biol 2016; 17: 13. doi: 10.1186/ s13059-016-0881-8.
3. Morey M, Fernández-Marmiesse A, Castiñeiras D et al. A glimpse into past, present, and future DNA sequencing. Mol Genet Metab 2013; 110(1–2): 3–24. doi: 10.1016/ j.ymgme.2013.04.024.
4. Liu L, Li Y, Li S et al. Comparison of next-generation sequencing systems. J Biomed Biotechnol 2012; 2012: 251364. doi: 10.1155/ 2012/ 251364.
5. Sanger F, Air GM, Barrell BG et al. Nucleotide sequence of bacteriophage φX174 DNA. Nature 1977; 265(5596): 687–695. doi: 10.1038/ 265687a0.
6. Heather JM, Chain B. The sequence of sequencers: the history of sequencing DNA. Genomics 2016; 107(1): 1–8. doi: 10.1016/ j.ygeno.2015.11.003.
7. Wolf JBW. Principles of transcriptome analysis and gene expression quantification: an RNA-seq tutorial. Mol Ecol Resour 2013; 13(4): 559–572. doi: 10.1111/ 1755-0998.12109.
8. Faktor J, Michalova E, Bouchal P. p-SRM, SWATH a HRM – cílené proteomické přístupy na hmotnostním spektrometru TripleTOF 5600+ a jejich aplikace v onkologickém výzkumu. Klin Onkol 2014; 27 (Suppl 1): 110–115. doi: 10.14735/ amko20141S110.
9. Ewing B, Green P. Base-calling of automated sequencer traces using phred. II. Error probabilities. Genome Res 1998; 8(3): 186–194.
10. Babraham Bioinformatics. FastQC: a quality control tool for high throughput sequence data. [online]. Available from: http:/ / www.bioinformatics.babraham.ac.uk/ projects/ fastqc.
11. Dai M, Thompson RC, Maher C et al. NGSQC: cross-platform quality analysis pipeline for deep sequencing data. BMC Genomics 2010; 11 (Suppl 4): S7. doi: 10.1186/ 1471-2164-11-S4-S7.
12. Yang IS, Kim S. Analysis of whole transcriptome sequencing data: workflow and software. Genomics Inform 2015; 13(4): 119–125. doi: 10.5808/ GI.2015.13.4.119.
13. Garber M, Grabherr MG, Guttman M et al. Computational methods for transcriptome annotation and quantification using RNA-seq. Nature Methods 2011; 8(6): 469–477. doi: 10.1038/ nmeth.1613.
14. Martin JA, Wang Z. Next-generation transcriptome assembly. Nat Rev Genet 2011; 12(10): 671–682. doi: 10.1038/ nrg3068.
15. Campbell PJ, Stephens PJ, Pleasance ED et al. Identification of somatically acquired rearrangements in cancer using genome-wide massively parallel paired-end sequencing. Nature Genet 2008; 40(6): 722–729. doi: 10.1038/ ng.128.
16. Wang Z, Gerstein M, Snyder M. RNA-Seq: a revolutionary tool for transcriptomics. Nat Rev Genet 2009; 10(1): 57–63. doi: 10.1038/ nrg2484.
17. Góngora-Castillo E, Buell CR. Bioinformatics challenges in de novo transcriptome assembly using short read sequences in the absence of a reference genome sequence. Nat Prod Rep 2013; 30(4): 490–500. doi: 10.1039/ c3np20099j.
18. Li H, Durbin R. Fast and accurate short read alignment with Burrows-Wheeler transform. Bioinformatics 2009; 25(14): 1754–1760. doi: 10.1093/ bioinformatics/ btp324.
19. Langmead B, Trapnell C, Pop M et al. Ultrafast and memory-efficient alignment of short DNA sequences to the human genome. Genome Biol 2009; 10(3): R25. doi: 10.1186/ gb-2009-10-3-r25.
20. Kim D, Pertea G, Trapnell C et al. TopHat2: accurate alignment of transcriptomes in the presence of insertions, deletions and gene fusions. Genome Biol 2013; 14(4): R36. doi: 10.1186/ gb-2013-14-4-r36.
21. Wu TD, Nacu S. Fast and SNP-tolerant detection of complex variants and splicing in short reads. Bioinformatics 2010; 26(7): 873–881. doi: 10.1093/ bioinformatics/ btq057.
22. De Bona F, Ossowski S, Schneeberger K et al. Optimal spliced alignments of short sequence reads. Bioinformatics 2008; 24(16): 174–180. doi: 10.1093/ bioinformatics/ btn300.
23. De Wit P, Pespeni MH, Ladner JT et al. The simple fool’s guide to population genomics via RNA-Seq: an introduction to high-throughput sequencing data analysis. Mol Ecol Resour 2012; 12(6): 1058–1067. doi: 10.1111/ 1755-0998.12003.
24. McKenna A, Hanna M, Banks E et al. The Genome Analysis Toolkit: a MapReduce framework for analyzing next-generation DNA sequencing data. Genome Res 2010; 20(9): 1297–1303. doi: 10.1101/ gr.107524.110.
25. Koboldt D, Zhang Q, Larson D et al. VarScan 2: Somatic mutation and copy number alteration discovery in cancer by exome sequencing. Genome Res 2012; 22(3): 568–576. doi: 10.1101/ gr.129684.111.
26. Li H, Handsaker B, Wysoker A et al. The sequence alignment/ map format and SAMtools. Bioinformatics 2009; 25(16): 2078–2079. doi: 10.1142/ S0219720015500250.
27. Yu X, Sun S. Comparing a few SNP calling algorithms using low-coverage sequencing data. BMC bioinformatics 2013; 14: 274. doi: 10.1186/ 1471-2105-14-274.
28. Recamo.cz. TransPEM (Software for Translation sequence into peptide based on mutation information). [online]. Dostupné z: https:/ / www.recamo.cz/ cz/ software/ transpem/ .
29. Ning K, Fermin D, Nesvizhskii A. Computational analysis of unassigned high-quality MS/ MS spektra in proteomic data sets. Proteomics 2010; 10(14): 2712–2718. doi: 10.1002/ pmic.200900473.
30. Sahin U, Derhovanessian E, Miller M et al. Personalized RNA mutanome vaccines mobilize poly-specific therapeutic immunity against cancer. Nature 2017; 547(7662): 222–226. doi: 10.1038/ nature23003.
31. Ott PA, Hu Z, Keskin DB et al. An immunogenic personal neoantigen vaccine for patients with melanoma. Nature 2017; 547(7662): 217–221. doi: 10.1038/ nature22991.
Labels
Paediatric clinical oncology Surgery Clinical oncologyArticle was published in
Clinical Oncology
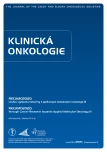
2019 Issue Supplementum 3
Most read in this issue
- Protein Ubiquitination Research in Oncology
- CRISPR-Cas9 as a Tool in Cancer Therapy
- Glycosylation as an Important Regulator of Antibody Function
- Uncommon EGFR Mutations in Non-Small Cell Lung Cancer and Their Impact on the Treatment